The article discusses the potential of Large Language Models (LLMs) in biomolecular text tasks, such as sequence analysis and natural language processing. These models have shown remarkable performance in various applications, including language translation, text summarization, and chatbots. However, there are challenges associated with using LLMs in the biosciences, primarily due to the lack of explicit manual labels and the need for techniques such as data mining and AI-assisted generation to extract and select relevant data.
To address these challenges, the authors propose a dataset of textual instructions that can be used to train LLMs in biomolecular text tasks. This dataset, known as "BioText," is designed to provide foundational models with the necessary guidance to comprehend and generate accurate text related to biomolecules. By using BioText, researchers can improve the performance of LLMs in these tasks, ultimately leading to more efficient and effective analysis and interpretation of biological data.
The article emphasizes the importance of ethical considerations when developing and using LLMs in the biosciences. This includes ensuring that access is regulated, monitoring usage patterns, establishing community oversight mechanisms, and promoting transparent reporting of any unintended outcomes or potential harmful use-cases.
In summary, the article presents a significant step forward in harnessing the power of LLMs for biomolecular text tasks while prioritizing ethical considerations. By providing a dataset of textual instructions and emphasizing the need for caution and diligence in their application, the authors demonstrate the potential of LLMs to transform the field of biosciences.
Quantitative Biology, Quantitative Methods
Harnessing AI for Biological Data Analysis: Efficient Generation of Q&A Pairs
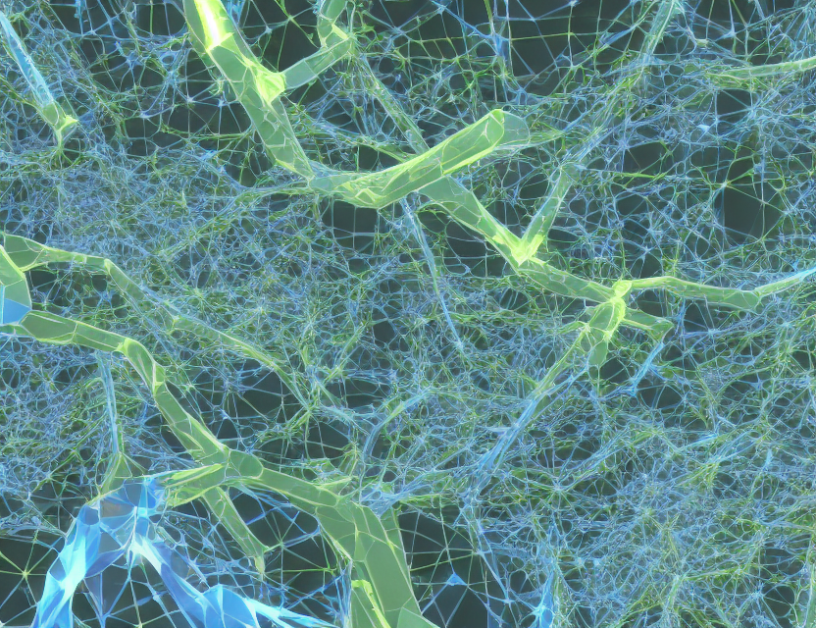