Energy disaggregation is a complex task that involves separating individual appliance energy consumption from the total power consumption data. Traditional methods rely on smart meters to collect aggregated data, but with the rise of edge/IoT devices, end-users can now have more autonomy by receiving actionable insights into their energy consumption. Graph Signal Processing (GSP) is a promising algorithm that can handle noisy data and deal with different sampling rates, making it efficient and reliable. However, there are still challenges to overcome, such as the robustness of the algorithm and the ability to deal with incomplete training sets.
Machine learning (ML) algorithms have shown good outcomes in energy disaggregation, including Random Forest and KNN classifier. However, labelled datasets are difficult to collect as they require a lot of resources and time. Researchers have had to manually label detected events according to occupants’ written diaries in one study, which can be time-consuming and costly.
In summary, energy disaggregation is crucial for energy management, and GSP and ML algorithms are promising approaches. However, there are still challenges to overcome, and collecting labelled datasets is a significant issue that needs to be addressed. By understanding these complex concepts and the challenges involved, we can demystify energy disaggregation and unlock its full potential for efficient energy management.
Electrical Engineering and Systems Science, Systems and Control
Energy Disaggregation: Dataset Collection Challenges and Opportunities
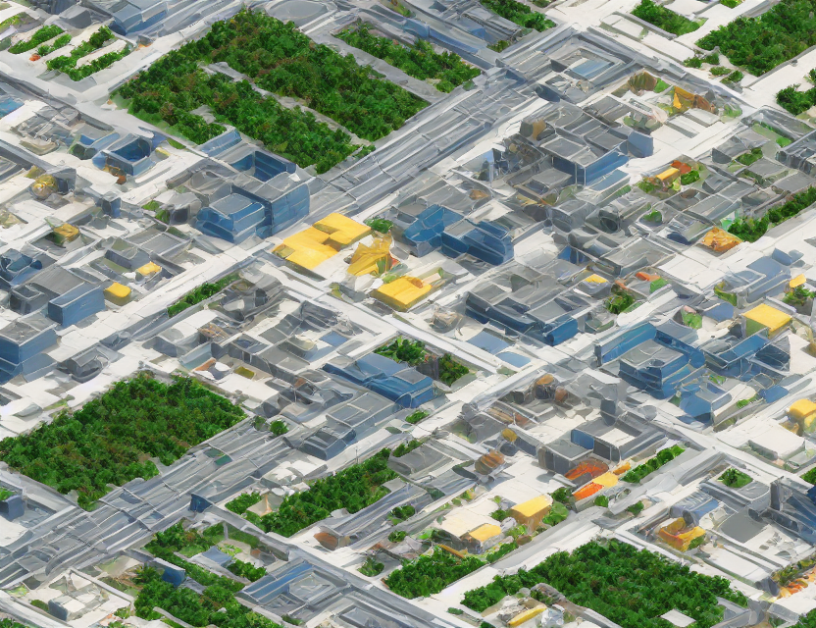