Computational biology has seen a surge of new machine learning (ML) architectures in recent years, each with its unique strengths and weaknesses. These architectures are designed to improve accuracy, speed, transferability, and data efficiency. Some mirror the symmetries of physical systems, while others incorporate limited amounts of physics into their models.
Imagine a Lego castle with different rooms representing different machine learning architectures. Each room has its own unique features and functions, but they all work together to create a comprehensive and accurate model of the castle. Some rooms are designed for speed and efficiency, while others prioritize accuracy and transferability.
One approach is to mirror the symmetries of the physical system, like a translational or rotational symmetry. This means that the machine learning architecture has the same properties under these symmetries, ensuring accurate predictions. Think of it like a Lego castle with identical parts on each side, maintaining a perfect balance and symmetry.
Another approach is to incorporate physics directly into the model. This can include terms for Coulomb interactions, dispersion, and nuclear repulsion. It’s like adding special features to the Lego castle, such as a drawbridge or a moat, to better represent real-world physics.
But why stop there? Some architectures take it even further by starting with a semi-empirical quantum chemistry method and training a machine learning model to correct for its flaws and improve accuracy. It’s like building a Lego castle from a blueprint, using the blueprint to add details and features that make it more realistic.
These new architectures provide different tradeoffs between speed, accuracy, and range of applicability. They are like different rooms in a castle, each with its unique purpose and function. The key is to choose the right room for the task at hand, depending on what’s most important.
In summary, recent advances in machine learning architectures have provided new tools for computational biology, allowing researchers to create more accurate models of complex systems. These architectures are like different rooms in a castle, each with its unique features and functions, but they all work together to create a comprehensive and accurate model of the system. By understanding these different approaches and their tradeoffs, researchers can choose the right tools for the task at hand and make significant progress in the field.
Researchers Unite to Advance Computational Chemistry and Biochemistry
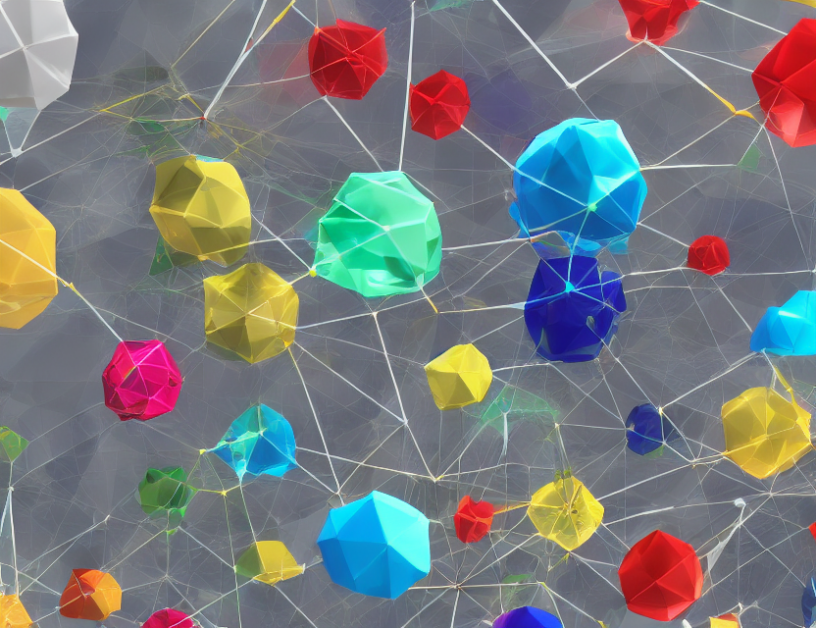