In this article, we delve into the exciting realm of artificial intelligence (AI) and its application in accelerator design. Accelerators are crucial components in various scientific research fields, including particle physics, materials science, and drug discovery. Traditionally, these accelerators are designed using trial-and-error methods, which can be time-consuming and costly. However, recent advancements in AI have enabled the development of neural network-based models that can efficiently and effectively design accelerators.
The article begins by introducing the context of scientific user facilities, highlighting the significance of accelerator design in various fields. The authors explain how AI has revolutionized the field of accelerator design by enabling the creation of neural network-based models that can simulate and optimize accelerator performance with unprecedented accuracy.
To comprehend how these models work, the authors break down the concepts into simpler terms. They explain that traditional accelerators are designed using a combination of physics and engineering principles. However, these designs often result in suboptimal performance due to limitations in the available computational resources. On the other hand, AI-powered neural network models can simulate and optimize accelerator performance by learning from large datasets and identifying patterns that were previously unknown.
The authors then delve into the specifics of how these neural networks are trained and optimized. They explain that the training process involves generating a dataset of simulated accelerator designs, using this dataset to train the neural network, and then optimizing the network by adjusting its parameters to improve its performance. The authors highlight the significance of careful hyperparameter tuning in this process, as improper tuning can result in suboptimal model performance.
Next, the authors discuss the various applications of these AI-powered accelerator designs. They explain that these models can be used to optimize accelerator performance for a wide range of scientific research fields, including particle physics, materials science, and drug discovery. The authors also highlight the potential for these models to enable novel accelerator designs that were previously impossible due to the limitations of traditional methods.
Finally, the authors emphasize the importance of collaboration between AI researchers and domain experts in the field of accelerator design. They explain that the success of these AI-powered models depends on the ability of both groups to understand each other’s perspectives and work together towards a common goal.
In conclusion, this article provides a comprehensive overview of how AI is transforming the field of accelerator design. By leveraging the power of neural networks, scientists can efficiently and effectively design accelerators that optimize performance for a wide range of scientific research fields. As this technology continues to evolve, we can expect to see novel accelerator designs that enable groundbreaking scientific discoveries and advancements.
Leveraging Normal Data for Anomaly Detection in Accelerators
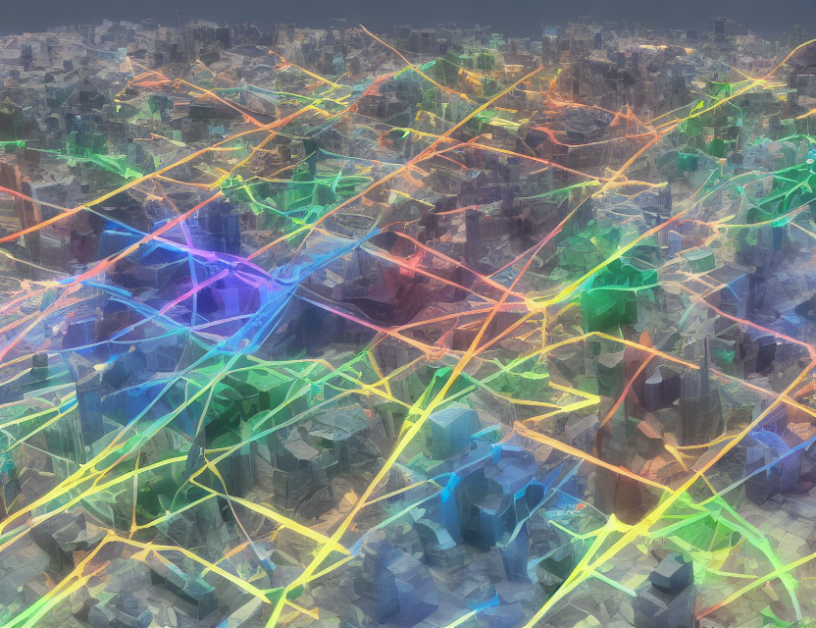