Deep learning segmentation is a cutting-edge technique used to analyze medical images, such as those obtained through magnetic resonance imaging (MRI). In this article, researchers evaluate the accuracy and efficiency of deep learning algorithms in real-time MRI segmentation. They compare the performance of various deep learning models, including U-Net and nnU-Net, against traditional computer vision techniques.
The study emphasizes the potential of deep learning to revolutionize medical imaging analysis by offering faster and more accurate results than previous methods. The authors highlight the importance of real-time segmentation in clinical settings, where timely diagnosis and treatment are crucial. They also stress the significance of validating these models on diverse datasets to ensure their generalizability.
To assess the performance of deep learning algorithms, the researchers use various metrics such as mean absolute error (MAE), mean squared error (MSE), peak signal-to-noise ratio (PSNR), and structural similarity index (SSIM). They demonstrate that deep learning models outperform traditional computer vision techniques in terms of segmentation accuracy and computational efficiency.
The authors also discuss the limitations of current deep learning approaches, including the need for large amounts of annotated data and the risk of overfitting due to model complexity. They emphasize the importance of addressing these challenges to further improve the quality of deep learning segmentation models.
In conclusion, this study provides a comprehensive assessment of deep learning segmentation for real-time MRI analysis, highlighting its potential to transform medical imaging analysis. The authors demonstrate the accuracy and efficiency of deep learning algorithms and emphasize the need for further research to overcome their limitations.
Electrical Engineering and Systems Science, Image and Video Processing
Deep Learning Segmentation for Real-Time CMR: An Assessment of Inter- and Intra-Observer Variability
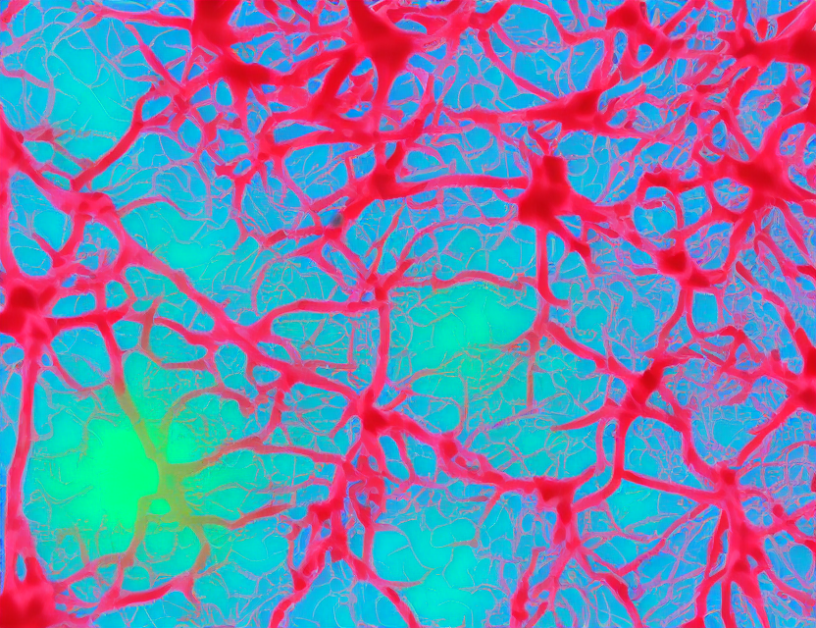