In this article, researchers propose a novel filtering method that addresses limitations in conventional techniques, specifically neural network-based methods and Koopman operator-based approaches. These techniques require significant computational resources and data to train the system model, making it challenging to analyze their performance. The proposed method leverages identification-based filtering, which focuses on estimating the system matrices, rather than relying solely on prediction errors. This approach offers two key advantages: (1) it provides a state-space realization up to a physical similarity transformation, and (2) it uses a closed-form relation to quantify the estimation performance.
The proposed method is designed to estimate the value of motor speed ˙θ and current i as accurately as possible. The researchers define a mean squared error (MSE) metric to measure the accuracy of these estimates, and an average squared estimation error (ASEE) metric to quantify the relationship between the estimation performance and sample complexity.
To illustrate their approach, the authors utilize pre-collected trajectories and demonstrate how the proposed method can accurately estimate ˙θ and i, even with limited data. They also show that their method outperforms traditional identification methods in terms of computational efficiency and accuracy.
In simple terms, this article presents a novel filtering technique that addresses limitations in existing methods. By focusing on estimating system matrices rather than prediction errors, the proposed approach offers more accurate and efficient filtering. The authors demonstrate the effectiveness of their method through real-world examples and provide insights into how it can be applied to practical applications.
Electrical Engineering and Systems Science, Systems and Control
Data-Driven Kalman Filtering: A Promising Approach to Enhance Performance
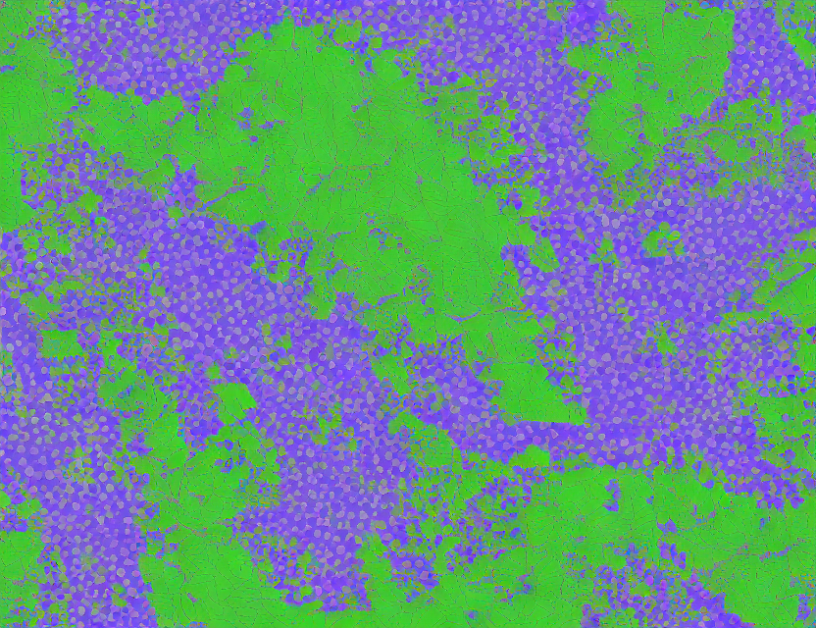