Autonomous vehicles (AVs) are becoming increasingly common on our roads, and their development relies heavily on game-theoretic approaches. These techniques allow AVs to make decisions based on the expected actions of other drivers, much like how humans drive. However, there are challenges that need to be addressed before these methods can be used in real-world scenarios.
The article discusses two main issues: game complexity and incomplete information. Game complexity refers to the difficulty of solving multiplayer games, which can make it hard for AVs to make decisions quickly and efficiently. Incomplete information is a problem because AVs lack knowledge about other drivers’ cost functions, making it challenging to predict their actions.
To overcome these challenges, the authors propose a potential game-based framework that relaxes the conditions on cost function design, making it applicable to more scenarios. This approach allows AVs to learn from experience and adapt to changing traffic patterns.
The article also highlights the importance of understanding the values of driver-specific parameters in cost functions, which can be achieved through data collection and analysis. By knowing these parameters, AVs can better predict other drivers’ behavior and make more informed decisions.
In summary, game-theoretic autonomous driving is a complex field that requires careful consideration of game complexity and incomplete information. While there are challenges to be addressed, the potential game-based framework proposed in this article offers a promising solution for AVs to learn from experience and adapt to changing traffic patterns. By understanding driver-specific parameters in cost functions, AVs can better predict other drivers’ behavior and make more informed decisions.
Electrical Engineering and Systems Science, Systems and Control
Modeling Multi-Agent Decision-Making for Interactive AVs
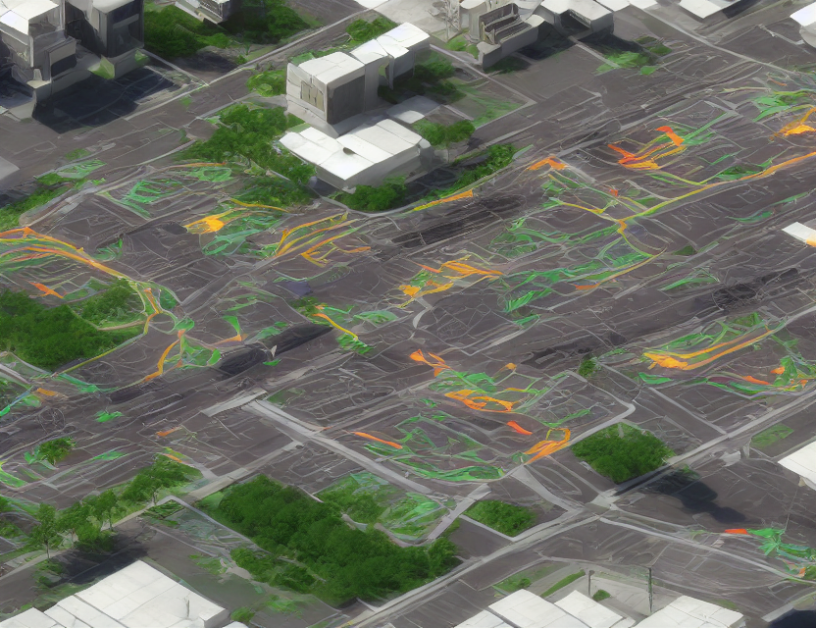