In the world of gene regulation, Transcription Factors (TFs) play a crucial role in controlling the expression of genes. TF cascades, which are linear or branching pathways of TFs, can help us understand how these genes are regulated. However, analyzing and predicting TF cascades is a complex task. To tackle this problem, researchers employed a machine learning approach called Graph ML, which creates a graph representation of the data and uses it to make predictions.
Firstly, the authors of the study constructed a dataset of TF cascades and analyzed it using Exploratory Data Analysis (EDA). This helped them understand the overall characteristics of the data and identify patterns that were not immediately apparent. Next, they employed different visualization techniques to better comprehend the relationships between TFs in the graph.
To create a more accurate prediction model, the authors restricted their analysis to univariate analysis, focusing on categorical variables – TFs. They then employed a link prediction algorithm to evaluate and rank the predicted links based on their scores. The purpose of creating a graph from the newly constructed TF cascades and enriched pathway dataset is to establish a more comprehensive and generalizable representation of TF cascades. This approach enables researchers to construct a foundational model that can be readily applied to various downstream tasks, such as Next TF prediction, TF classification, and Pathway prediction, among others.
In summary, the article presents a novel approach to analyzing and predicting TF cascades using Graph ML. By creating a comprehensive representation of TF cascades, researchers can gain insights into how genes are regulated and make predictions about future TF interactions. The use of graph-based methods is particularly useful in this context as it allows for the analysis of relationships between entities rather than sequences of states of a single entity.
Molecular Networks, Quantitative Biology
Network Analysis Reveals Key Transcription Factors in TF Cascades
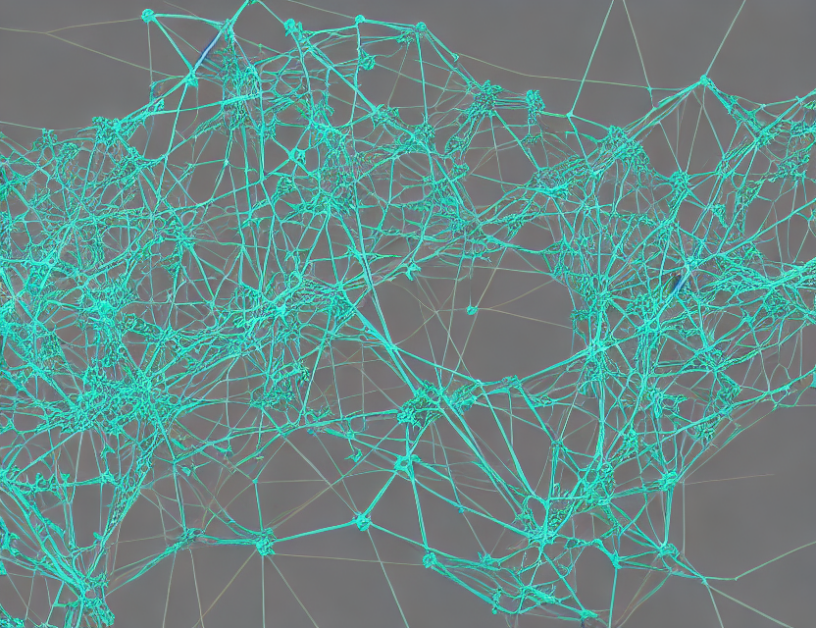