Phylogenetic networks are a way to represent evolutionary relationships between organisms, taking into account recombination and other reticulate events. Unlike phylogenetic trees, which have nodes with only two parents, nodes in phylogenetic networks can have multiple parents, making them more complex. Inference of phylogenetic networks from data is an important problem in computational biology, but the number of possible networks is very large, even for small numbers of reticulate nodes.
One approach to solving this problem is to use lower bounds on the distance between the true network and a given bounding tree or network. These lower bounds can be used to evaluate the quality of different networks and guide the search for the best one. However, computing these lower bounds can be computationally challenging, especially when dealing with large amounts of data.
The article discusses three types of lower bounds that have been proposed in the literature: TCLB0, TCLB1, and TCLB2. These bounds are based on different properties of phylogenetic networks, such as the tree-child number or the presence of certain types of subtrees. The article also compares these lower bounds with other upper bounds that have been proposed in the literature, including ALTS and PIRNs.
The authors demonstrate that TCLB0 can be easily computed for large data sets, while TCLB1 and TCLB2 require more complex algorithms that are not yet polynomial time. They also show that these lower bounds can be used to evaluate the quality of different networks and guide the search for the best one.
In summary, this article provides an overview of the state-of-the-art in computing lower bounds on the distance between a true phylogenetic network and a given bounding tree or network, with a focus on three types of lower bounds that have been proposed in the literature: TCLB0, TCLB1, and TCLB2. The article also compares these lower bounds with other upper bounds and demonstrates their usefulness in evaluating the quality of different networks.
Populations and Evolution, Quantitative Biology
Accurate Lower Bounds for Phylogenetic Tree-Child Reticulation
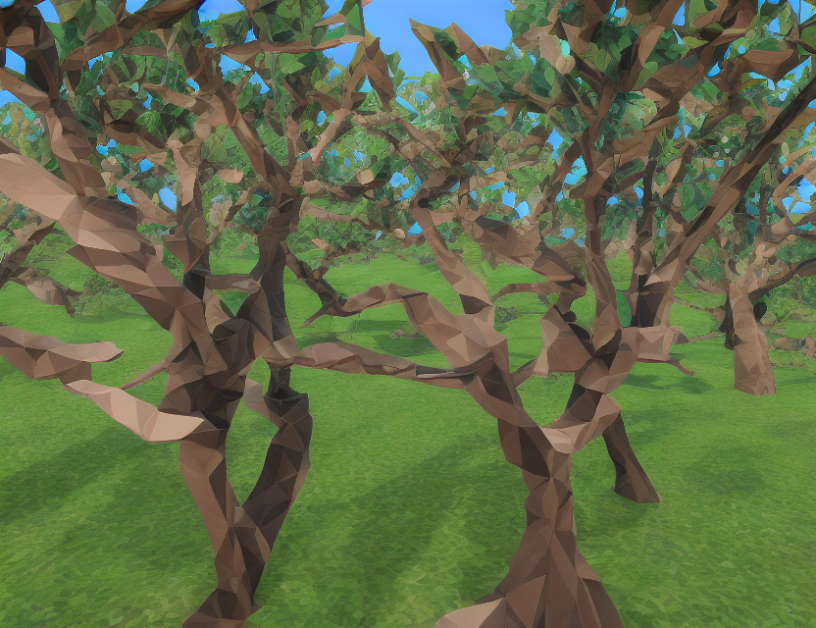