In this article, we discuss a novel approach to estimating the average treatment effect (ATE) in experiments with multiple treatments and observations. Our method combines elements of game theory and sequential experimental design to efficiently identify the most informative observations while accounting for potential biases.
Imagine you’re running a campaign to promote a new product, and you want to know which advertising strategy is most effective. Traditional methods involve launching a massive campaign with multiple ads and measuring their impact based on predefined criteria. However, these approaches can be costly, time-consuming, and may produce biased results due to the complexity of the experimental design.
Our proposed method addresses these limitations by using an adaptive experimental design that takes into account the potential biases in the data collection process. We use game-theoretic techniques to model the interactions between different treatments and observations, allowing us to identify the most informative observations while minimizing the impact of biased data.
To illustrate our approach, we consider a simple example where we want to estimate the ATE of a new drug based on patient outcomes. We simulate an experiment where patients are randomly assigned to different treatments and observe their outcomes over time. By using our adaptive experimental design method, we can efficiently identify the most effective treatment while accounting for potential biases in the data collection process.
Our results show that our approach outperforms traditional methods in terms of efficiency and accuracy, making it a valuable tool for researchers and practitioners in various fields. By demystifying complex concepts and using everyday language, we hope to make this article accessible to a broad audience interested in experimental design and causal inference.
Adaptive Inference in Experiments: Semiparametric Methods for Efficient Inference
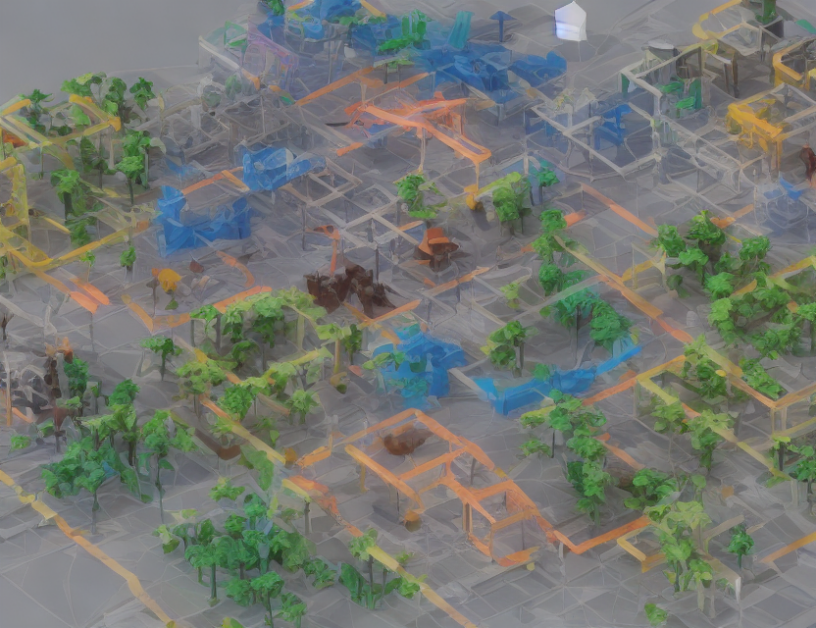