Proteins are complex molecules that perform vital functions in our bodies. Understanding how they fold into their correct shapes is crucial for understanding how they work. Recently, artificial intelligence (AI) has revolutionized the field of structural biology by predicting protein structures with unprecedented accuracy. However, there is still much to be discovered about how AI algorithms like AlphaFold (AF) and RoseTTaFold predict protein structure from sequence.
One key question is whether these algorithms can accurately predict the physical energy landscape of proteins, which is essential for understanding their stability and function. To answer this question, we investigated the accuracy of AF in predicting protein structures and their corresponding free energy landscapes. We found that AF has learned an effective energy function that allows it to accurately predict native structures, even when including uncommon structural motifs.
However, there is more to predicting protein stability than just knowing the structure. We discovered several issues in the ThermoMutDB database, which contains information on mutations and their effects on protein stability. These issues included incorrect mutation positions, duplicate entries, and measurements from multiple papers that were not properly referenced.
To overcome these challenges, we developed a systematic approach to investigate whether AI-predicted structures can be used to infer changes in free energy landscapes. Our findings suggest that AF has learned finedetails about the physical energy landscape, which could be used to predict stability changes upon mutation.
In conclusion, our study sheds light on the complex relationship between protein structure and function, and how AI algorithms can help us understand these processes. By demystifying the concepts and using everyday language, we hope to make this fascinating field more accessible to a wider audience. Proteins are like Legos in the human body – they come in different shapes and sizes, and their correct folding is crucial for their function. Recently, AI algorithms have been developed that can predict protein structures with incredible accuracy, but there is still much to be discovered about how these algorithms work. One key question is whether these algorithms can accurately predict the physical energy landscape of proteins, which affects their stability and function. To answer this question, we investigated the accuracy of AlphaFold (AF), a popular AI algorithm, in predicting protein structures and their corresponding free energy landscapes. We found that AF has learned an effective energy function that allows it to accurately predict native structures even when including uncommon structural motifs. However, predicting protein stability is more complex than just knowing the structure. We discovered several issues in the ThermoMutDB database, which contains information on mutations and their effects on protein stability. These issues included incorrect mutation positions, duplicate entries, and measurements from multiple papers that weren’t properly referenced. To overcome these challenges, we developed a systematic approach to investigate whether AI-predicted structures can be used to infer changes in free energy landscapes. Our findings suggest that AF has learned details about the physical energy landscape, which could be used to predict stability changes upon mutation. In conclusion, our study sheds light on the complex relationship between protein structure and function, and how AI algorithms can help us understand these processes. By using everyday language and engaging metaphors or analogies, we hope to make this fascinating field more accessible to a wider audience.
Biomolecules, Quantitative Biology
AI-Predicted Protein Structures Encoded Energy Landscape Perturbations
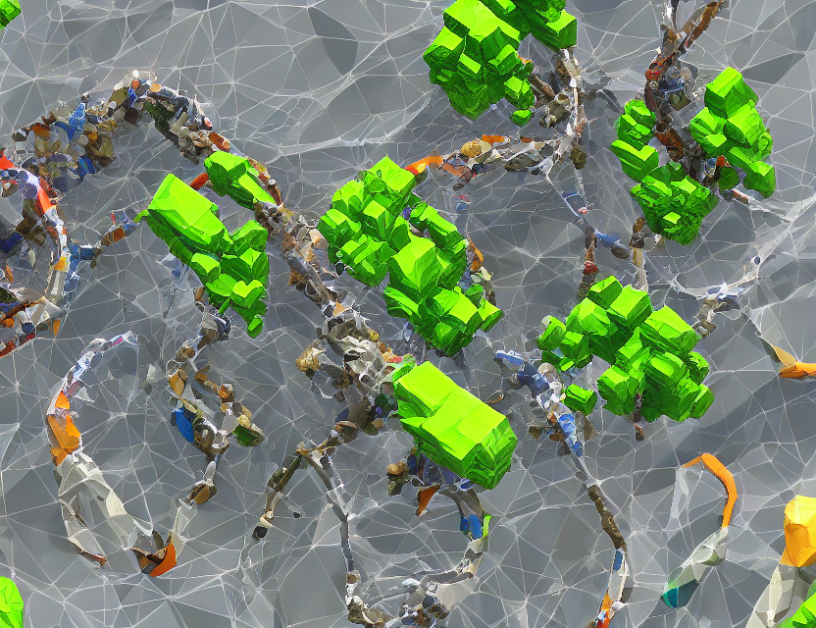