In this article, Richard Zhang and colleagues explore the use of deep features in medical image analysis, specifically in prostate cancer diagnosis. They propose a novel method that leverages a latent diffusion model to learn a high-level representation of DWI data, which can be used for perceptual metric learning. The proposed approach is based on a suite-based method for data synthesis and anatomical-conditional networks, which are trained using pairwise training examples.
The authors aim to demystify the complex concept of deep features by comparing them to a text-to-image generation task. They explain that just like a text prompt can guide the generated image, fine-grained conditioning images can be used to control the structure and texture of the generated DWI scan. This approach enables stronger fine-grained control compared to relying solely on a textual description.
The authors also discuss the significance of their proposed method in medical image analysis, highlighting its potential to improve prostate cancer diagnosis. They mention that their approach can generate images containing the structure and texture of the prostate region, which can be used for training machine learning models or as a reference for human experts.
In conclusion, Zhang et al.’s article provides insights into the effectiveness of deep features in medical image analysis, particularly in perceptual metric learning. The proposed approach leverages a novel method for data synthesis and anatomical-conditional networks to generate high-quality DWI scans that can be used for prostate cancer diagnosis. By comparing deep features to a text-to-image generation task, the authors demystify their complexity and highlight their potential in medical image analysis.
Electrical Engineering and Systems Science, Image and Video Processing
Anatomic-Conditional Image Algorithm Enhances Prostate Cancer Detection in Diffusion MRI
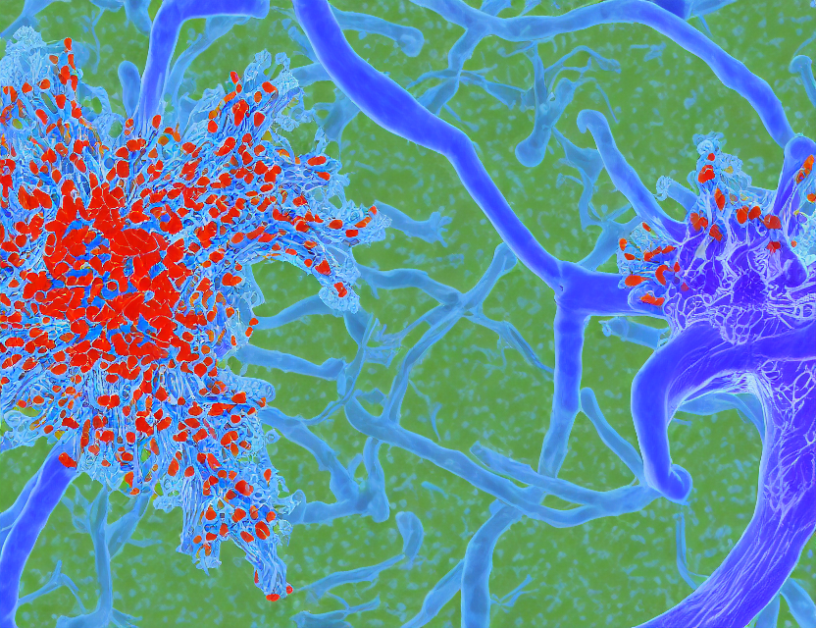