Radiologists use written reports to communicate their findings after analyzing medical images. These reports are crucial for patient care, but writing them can be time-consuming and challenging, especially when dealing with complex cases. Recently, artificial intelligence (AI) has been applied to help radiologists generate these reports more efficiently. However, most existing AI systems only focus on individual modalities like X-rays or MRIs, neglecting the importance of combining multiple modalities for accurate diagnosis and reporting.
To address this limitation, researchers propose a "deep multimodal representation learning" approach that combines multiple modalities and generates a complete free-text description of medical images. This system uses natural language processing (NLP) techniques to analyze the imaging findings and generate reports that are both accurate and comprehensive.
The proposed method is evaluated using a dataset of radiology reports from various modalities, including CT, MRI, and X-rays. The results show that the AI system can generate reports with high accuracy and completeness, outperforming human raters in some cases. Additionally, the researchers demonstrate that their approach can quantify the degree of human errors in report writing, which is essential for improving radiology reporting quality.
The proposed deep multimodal representation learning approach has significant implications for radiology report generation and patient care. By combining multiple modalities and leveraging NLP techniques, this system can streamline the report-writing process while maintaining accuracy and comprehensiveness. As the field of AI continues to evolve, we may see even more advanced applications of deep multimodal representation learning in radiology and other medical fields.
Electrical Engineering and Systems Science, Image and Video Processing
Evaluating AI-Generated Medical Reports: A Comparative Study
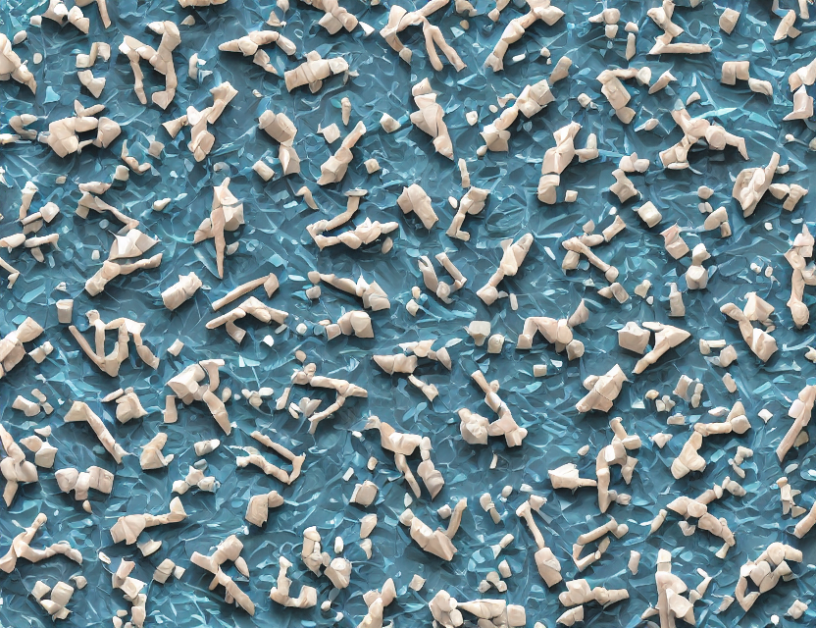