In this article, we discuss a new approach to solving partial differential equations (PDEs) in electromagnetism called domain decomposition methods. These methods break down a complex problem into smaller, manageable parts and solve them independently. However, when dealing with highly heterogeneous problems, it can be challenging to obtain accurate solutions without additional techniques.
One such technique is the use of spectral coarse spaces, which are like a series of filters that help remove unwanted signals from the solution. By using these filters, we can improve the accuracy and robustness of the solution, making it more reliable and less prone to errors.
To understand how this works, imagine a complex problem as a big jar of mixed-up ingredients. Just like cooking, we need to separate the different components of the problem to make sense of it. By using domain decomposition methods, we can break down the problem into smaller, more manageable parts that are easier to handle.
However, just like a bad recipe can lead to an uneven flavor, a poorly designed preconditioner can result in an unstable solution. This is where spectral coarse spaces come in. They act as a secret ingredient, helping us to remove any unwanted flavors and produce a more balanced dish.
In summary, domain decomposition methods are like a recipe book for solving complex problems. By breaking down the problem into smaller parts, we can make it easier to handle. However, we need to add an extra step, just like adding a secret ingredient to a recipe, to ensure that our solution is accurate and robust. This is where spectral coarse spaces come in – they help us remove unwanted signals and produce a more reliable solution.
Mathematics, Numerical Analysis
Reliable Comparison of Nearly Singular Helmholtz Solvers via Splitting and Preconditioning
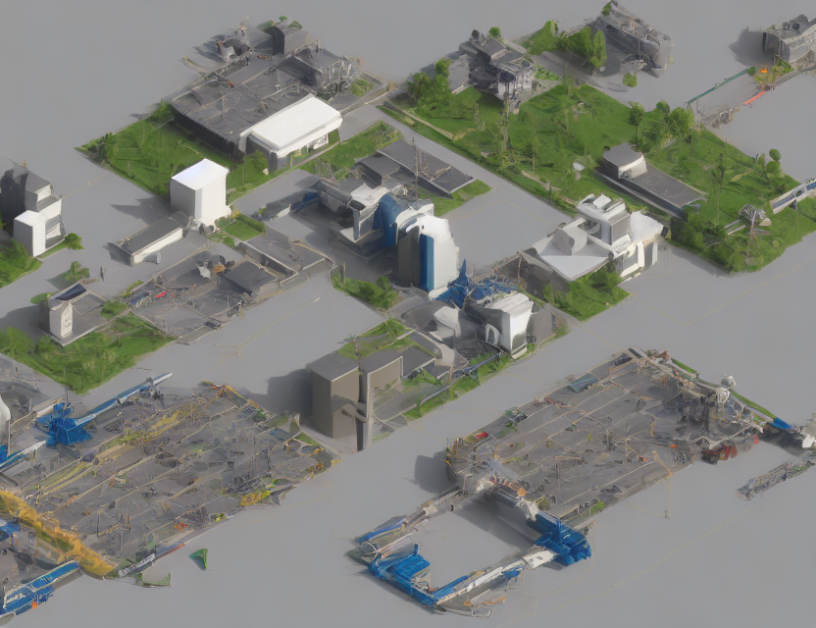