Alignment-free methods have gained popularity due to their faster computation time. One such method is Scaled Gaussian Metric (SGM), which extracts Gaussian invariants from protein backbones using knot theory to create a 30-dimensional vector representation of protein structures. Another alignment-free approach is Secondary Structure Element Footprint (SSEF), which counts the frequency of secondary structure triples to create a 1500-dimensional vector representation.
Shannon entropy, commonly used to evaluate the amount of information in a system, is also calculated for each descriptor in GraSR and FoldExplorer, two popular protein structure representation learning methods. The results show that FoldExplorer has learned a more comprehensive representation of the protein structure space, with higher Shannon entropy than GraSR.
Conclusion: Protein structure representation learning is an essential tool for analyzing protein structures in various biological applications. Various methods have been developed to represent protein structures in a simplified form while preserving their essential information. Alignment-free methods have gained popularity due to their faster computation time. In conclusion, this review summarizes the current state of protein structure representation learning and highlights its potential for advancing drug discovery and other biological applications.
Biomolecules, Quantitative Biology
Trade-Offs in Protein Representation Learning: Evaluating Methods for Drug-Protein Interactions
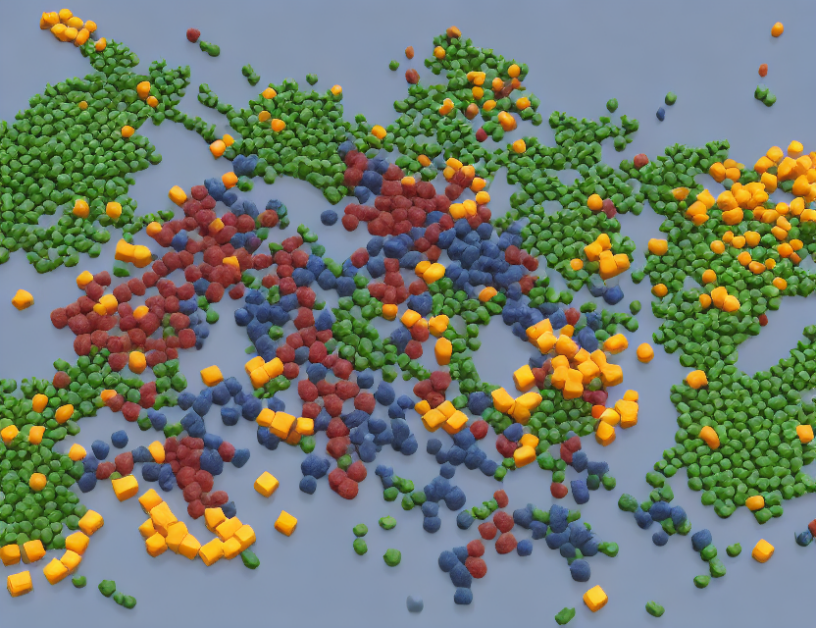