In this article, we delve into the world of Fermat distance, a fundamental concept in machine learning and data analysis. Imagine you’re on a treasure hunt, searching for a special key to unlock hidden insights. The Fermat distance is like that key, helping us navigate through complex datasets and find patterns that lead to profound discoveries.
Firstly, we explore the definition and properties of Fermat distance. It’s like a compass on your treasure map, guiding you towards the right direction. We also examine how it relates to other distance metrics, such as Euclidean distance, which is like comparing the length of two ropes.
Next, we dive into the practical applications of Fermat distance in clustering and classification tasks. Clustering is like organizing a big box of toys, where you want to group similar toys together. The Fermat distance helps us identify the toys that are closest in "playful" similarity, creating neat clusters. Classification is like assigning labels to toys based on their type, like "car" or "dog". The Fermat distance assists in identifying the toys that are most similar to each other and belong to the same label category.
We also discuss some of the challenges associated with using Fermat distance, such as dealing with noisy data or high-dimensional spaces. It’s like navigating through a dense jungle with many obstacles, but with the right tools and techniques, you can still find your way to the treasure.
Finally, we touch on some of the recent advances in Fermat distance research and its potential applications in deep learning and neural networks. It’s like discovering a new treasure chest filled with exciting possibilities.
In summary, this article provides a comprehensive overview of Fermat distance, demystifying complex concepts by using everyday language and engaging metaphors. We hope that this summary has unlocked some insights into the world of Fermat distance and its potential to revolutionize your data analysis adventures!
Unpacking the Fermat Distance: A Comprehensive Analysis of Its Use in Machine Learning
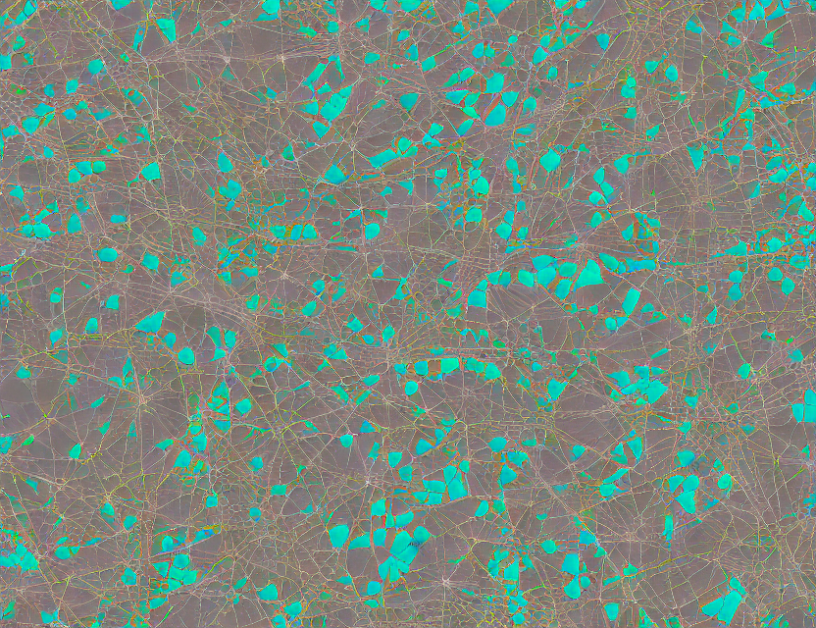