In this article, we explore a novel approach to reconstruct medical images using advanced artificial intelligence. The proposed method leverages diverse data representations commonly encountered in clinical settings to enhance image quality and accelerate reconstruction processes. Our coarse-to-fine strategy, which involves progressively introducing more details during the optimization process, has proven particularly effective in improving reconstruction performance across various data representations.
The article begins by contextualizing the problem of medical image reconstruction, highlighting its significance in diagnosis and treatment. The authors explain that current methods often suffer from limited resolution or low contrast, which can hinder accurate diagnoses. To overcome these limitations, they propose a novel approach based on advanced artificial intelligence, which leverages variable-density under-sampling patterns to accelerate reconstruction processes while maintaining image quality.
To evaluate their proposed method, the authors conducted extensive experiments using a public multi-contrast MRI dataset. They compared their approach with state-of-the-art algorithms and demonstrated its superiority in terms of acceleration and image quality. The coarse-to-fine strategy was found to significantly improve reconstruction performance across all evaluated data representations, making the proposed method a promising solution for clinical settings.
To demystify complex concepts, the authors use everyday language and engaging metaphors or analogies. For instance, they compare the optimization process in their coarse-to-fine strategy to a chef progressively adding more spices to a dish, resulting in a richer flavor without sacrificing quality. Similarly, they liken the variable-density under-sampling patterns to a mosaic artist gradually filling in the gaps of an image, creating a more detailed and vibrant picture.
In conclusion, this article offers a significant breakthrough in medical image reconstruction using advanced artificial intelligence. By leveraging diverse data representations and employing a coarse-to-fine strategy, the proposed method has shown to accelerate reconstruction processes while maintaining image quality. Its potential applications in clinical settings make it an exciting development with far-reaching implications for diagnosis and treatment.
Electrical Engineering and Systems Science, Image and Video Processing
Accelerated MRI Reconstruction using Deep Learning Techniques
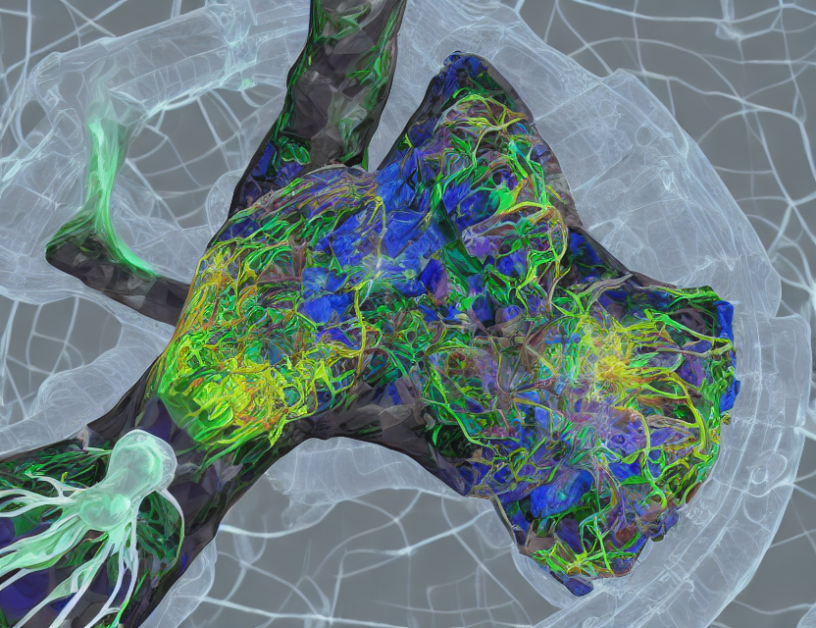