Semantic communications, which aim to transmit information more accurately, have gained significant attention in recent years due to advancements in artificial intelligence (AI). This field can be divided into two types: data-reconstruction-oriented and task-oriented semantic communications. The former tries to recreate the original data using semantic features, while the latter focuses on directly performing tasks using these features.
Current research has shown that using complex representations in neural networks (CvCNN) can improve the performance of image and signal processing tasks. However, CvCNN requires more complicated computations and longer computation time than traditional convolutional neural networks (CNN). Despite this, CvCNN offers several advantages, including better generalization, faster learning, and noise-robust memory mechanisms.
Another promising technology for semantic communications is metasurface-based modulation (MIM), which utilizes a programmable metasurface called a reconfigurable intelligent surface (RIS). RISs can manipulate the phase of an incident wave and are particularly useful in reducing the effect of noise on communication signals.
While RIS-based semantic communications offer many advantages, there are also some challenges to consider. For example, the propagation between two RIS layers is influenced by both layers, which can lead to complex computations and longer computation time. Moreover, it is important to carefully consider the parameters of CvCNN when using RISs to avoid oversimplifying the system.
In summary, RIS-based semantic communications offer a promising solution for improving the accuracy of information transmission by leveraging the advantages of complex representations and MIM technology. However, careful consideration must be given to the challenges associated with these technologies to ensure their optimal use in practical applications.
Electrical Engineering and Systems Science, Signal Processing
AI-Powered Semantic Communications: A Promising Future for Efficient Data Reconstruction
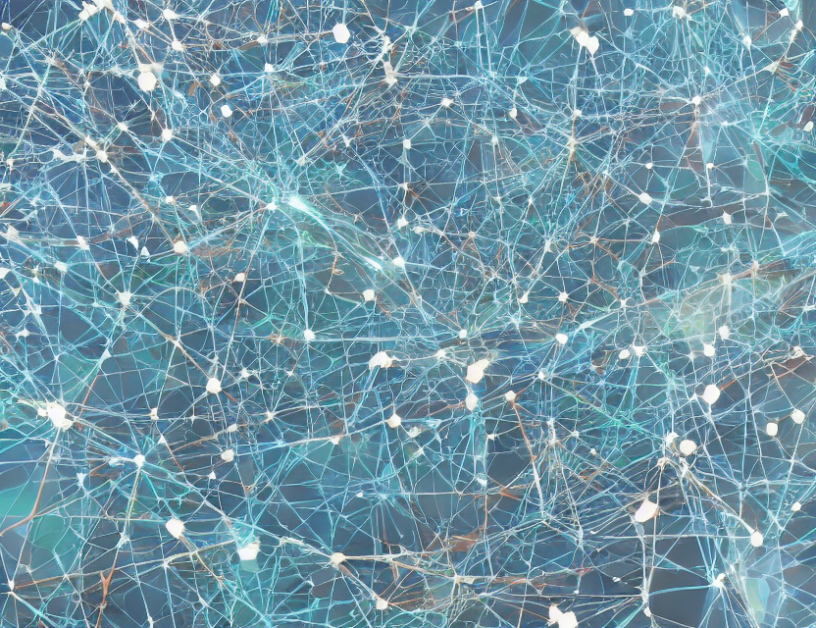