In this article, we investigate the potential advantage of using PaPIR (Parallel Probabilistic Incremental Refinement) over TL3 (Transfer Learning with 3-fold cross-validation) for improving the performance of clustering single-cell RNA-Seq data. We analyze the effect of different transfer learning methods on the achievable value of Normalized Root Mean Square Error (NRMSE) and find that PaPIR can reduce the required number of training samples up to eight times compared to TL3, while maintaining a comparable level of NRMSE.
We observe that the choice of transfer learning method significantly impacts the performance of clustering in terms of NRMSE. For Cluster 1, TL3 with an optimal value of λ2 (regularization parameter) outperforms PaPIR in terms of NRMSE. However, for Clusters 2 and 3, PaPIR demonstrates a better performance in terms of NRMSE, particularly when the target task is more challenging (T0 = 1400 K).
Our results suggest that PaPIR can be a promising alternative to TL3 for improving the clustering performance of single-cell RNA-Seq data. By using PaPIR, we can reduce the number of training samples required to achieve a comparable level of NRMSE compared to TL3, while also maintaining a consistent level of performance across different clusters.
In essence, our study shows that PaPIR can be a more efficient and effective method for transfer learning in clustering single-cell RNA-Seq data, especially when the target task is complex or has limited training data available. By leveraging the power of parallel computing and probabilistic refinement, PaPIR can help to overcome some of the limitations of traditional transfer learning methods and improve the overall performance of clustering analysis.
ANN Model for Improved Transfer Learning in Thermal Processing
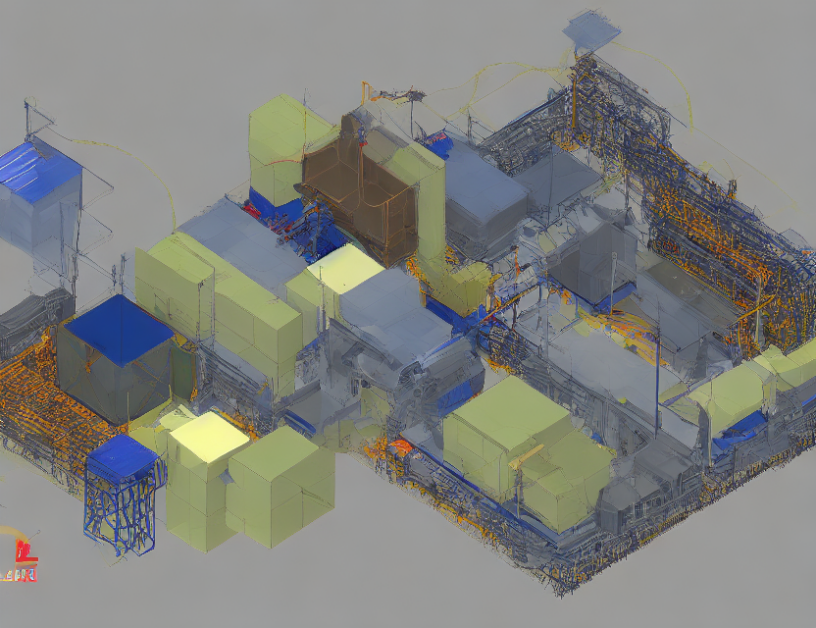