In this article, we explore how to use Bayesian model selection to choose the best causal graph for a given dataset, taking into account interventions on the graph. We start by explaining that causal graphs are used to represent complex relationships between variables in a system, and that Bayesian model selection is a way to choose the best statistical model for a given dataset based on prior knowledge of the underlying distribution.
We then delve into the specifics of causal graph model selection, noting that this task involves finding the DAG (directed acyclic graph) that is most likely to have generated the observed data, given some assumptions about the distribution of the data. We explain that there are two types of interventions in causal graphs: hard and soft. A hard intervention removes an edge from the graph entirely, while a soft intervention modifies the relationship between nodes without completely destroying it.
The article then discusses how to apply Bayesian model selection to this setting, using a framework called Bayesian model selection for DAGs (BMS-DAG). This method involves constructing a set of possible DAGs that could have generated the observed data, and then using a Bayesian approach to select the most likely DAG from this set. We explain that the selection process is based on a compatibility requirement between the selected DAG and the interventions applied to the graph.
Finally, we discuss some of the challenges associated with applying BMS-DAG to real-world data, such as dealing with incomplete data and handling multiple interventions. The article concludes by highlighting the importance of using Bayesian model selection for causal graph selection in the presence of interventions, as it allows for a more flexible and accurate analysis of complex systems.
In summary, this article provides a detailed overview of how to use Bayesian model selection to choose the best causal graph for a given dataset when interventions are taken into account. It explains the key concepts and techniques involved in this process and highlights some of the challenges associated with applying BMS-DAG to real-world data. The article provides a valuable resource for researchers and practitioners interested in using Bayesian model selection for causal graph selection in complex systems.
Detecting Differences in Causal Graphs Under Interventions: A Comparative Study
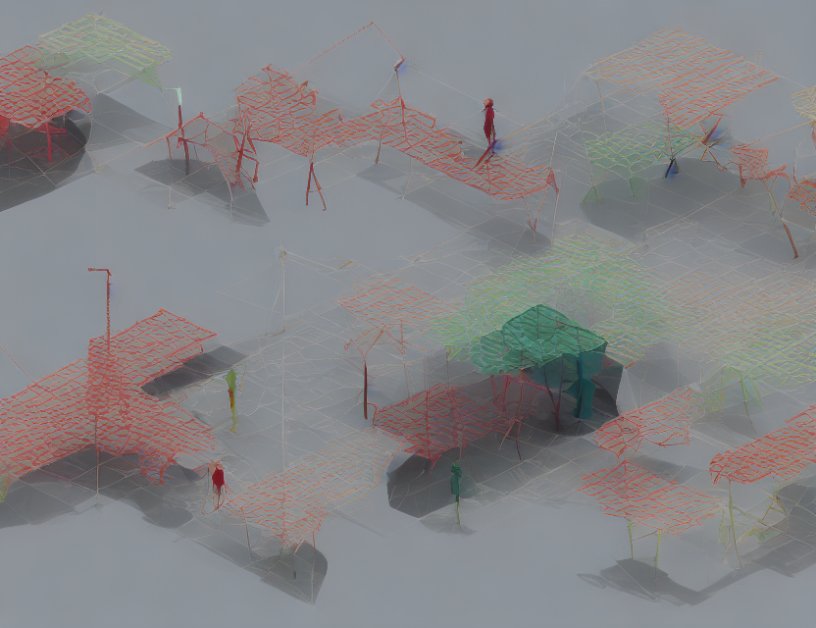