In this article, the authors propose a new deep learning framework called Monotone-Non-Monotone MOL (MnM-MOL) for solving inverse problems in imaging. The key innovation of MnM-MOL is its ability to learn potentially non-monotone functions, which can lead to improved performance compared to traditional monotone frameworks.
Imagine you are trying to solve a complex puzzle where you have a few pieces with different shapes and colors. A monotone framework would force the puzzle pieces to fit together in a specific way, without considering any non-monotone possibilities. However, MnM-MOL allows the puzzle pieces to fit together in different ways, even if they are not monotone. This flexibility can lead to a more accurate solution.
The proposed MnM-MOL scheme has several theoretical guarantees of uniqueness, convergence, and robustness similar to traditional MOL frameworks. Additionally, the relaxation of the constraint translates to improved performance. Think of it like loosening a tight grip on a puzzle piece – it may allow for more creative solutions that can fit together better.
The authors demonstrate the effectiveness of MnM-MOL in various imaging applications, including magnetic resonance imaging (MRI) and computed tomography (CT). They show that MnM-MOL outperforms traditional frameworks in these applications by leveraging its ability to learn non-monotone functions.
In summary, MnM-MOL is a powerful deep learning framework for solving inverse problems in imaging. By allowing for potentially non-monotone functions, it can lead to more accurate and creative solutions than traditional monotone frameworks. Its theoretical guarantees of uniqueness, convergence, and robustness make it a reliable choice for a wide range of applications.
Electrical Engineering and Systems Science, Image and Video Processing
Monotone Neural Networks for Image Recovery
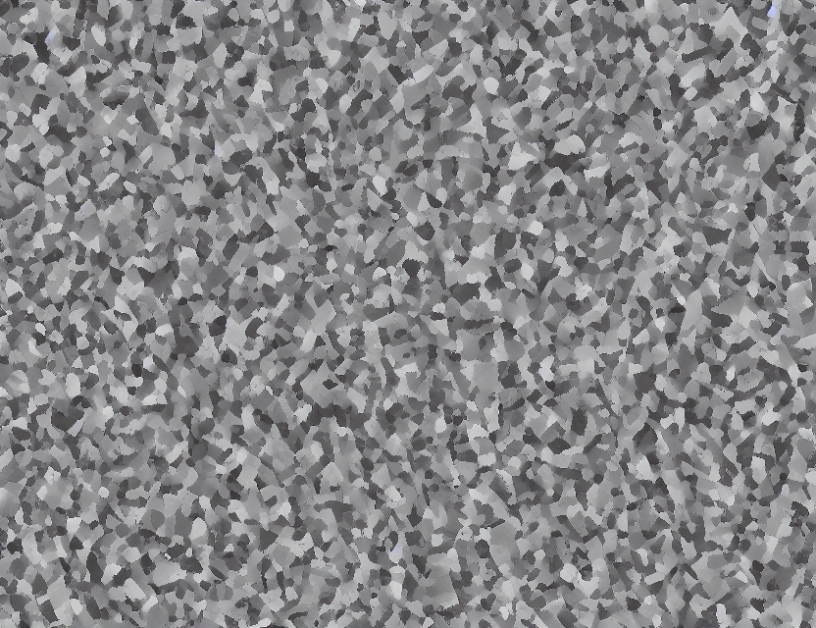