Medical image segmentation is a crucial task in healthcare, which involves identifying and labeling different objects or structures within medical images. Recently, researchers have been exploring the use of Vision Transformers (ViTs) for this task, as they offer several advantages over traditional computer vision techniques.
To understand how ViTs work, let’s first consider the limitations of traditional convolutional neural networks (CNNs). CNNs are good at analyzing small regions of an image but struggle with longer-range dependencies. This is where ViTs come in – they use self-attention mechanisms to process sequences of patches from an image, allowing them to capture longer-range dependencies more effectively.
ViTs have been shown to achieve state-of-the-art performance in various computer vision tasks, including medical image segmentation. By combining the strengths of both CNNs and ViTs, researchers have created hybrid models that leverage the best of both worlds. These models have demonstrated even better performance than their individual components, demonstrating the potential of Vision Transformers in medical image segmentation.
In summary, Vision Transformers are a powerful tool for medical image segmentation, offering improved performance and capabilities compared to traditional computer vision techniques. By leveraging self-attention mechanisms and combining them with other architectures, researchers have created hybrid models that can better capture longer-range dependencies and improve overall accuracy.
Electrical Engineering and Systems Science, Image and Video Processing
Vision Transformers for Automated Skin Lesion Segmentation
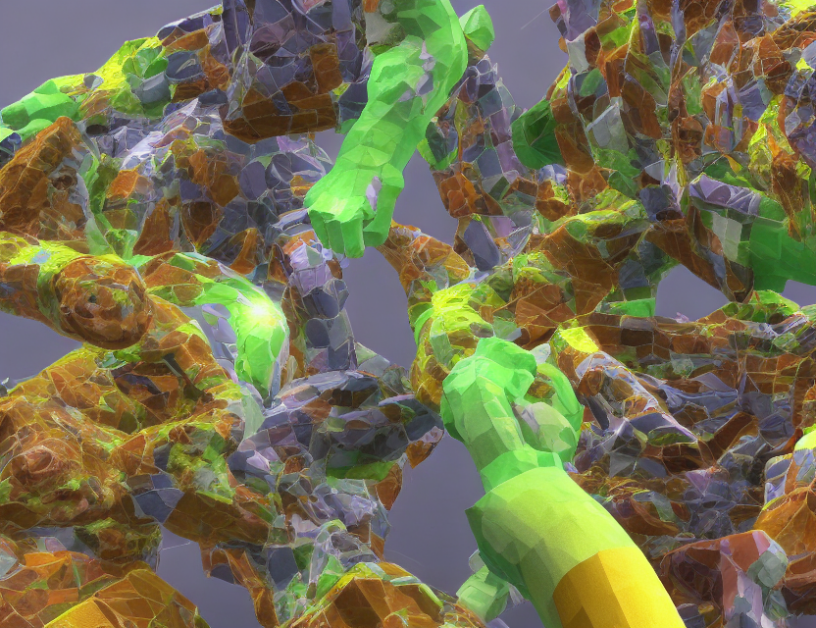