In this paper, we propose a new method for multi-modal data analysis called average-consensus matrix factorization (ACMF). Our approach leverages the idea of consensus matrix factorization, which is commonly used in single-modal data analysis. However, ACMF differs by considering multiple modalities simultaneously and incorporating an average factorization to better capture the relationships between the modalities.
Imagine you have a big box full of different toys, each with its own unique features and properties. To understand these toys better, you might want to use a tool that can help you identify patterns and relationships between them. This is where ACMF comes in – it’s like a special kind of magnifying glass that looks at all the toys together and finds common patterns and trends that might not be immediately visible.
The key insight behind ACMF is that the modalities are related, but they also have some differences. By finding a balance between these similarities and differences, ACMF can create a more accurate representation of the data than any single modality could on its own. This is achieved by using an average factorization, which smooths out the variations between the modalities to reveal underlying patterns that would otherwise be lost.
ACMF has many potential applications in fields such as computer vision, natural language processing, and recommender systems. For example, it could be used to improve image recognition algorithms by combining information from multiple images, or to develop more personalized recommendation systems by analyzing multiple sources of user data.
In summary, ACMF is a powerful tool for multi-modal data analysis that leverages the idea of consensus matrix factorization to capture complex relationships and patterns in the data. By considering multiple modalities simultaneously and incorporating an average factorization, ACMF can create more accurate representations of the data than any single modality could on its own. Its applications have the potential to revolutionize fields such as computer vision, natural language processing, and recommender systems.
Electrical Engineering and Systems Science, Systems and Control
Data-Driven Predictive Control: Uncertainty Estimation and Confidence Regions
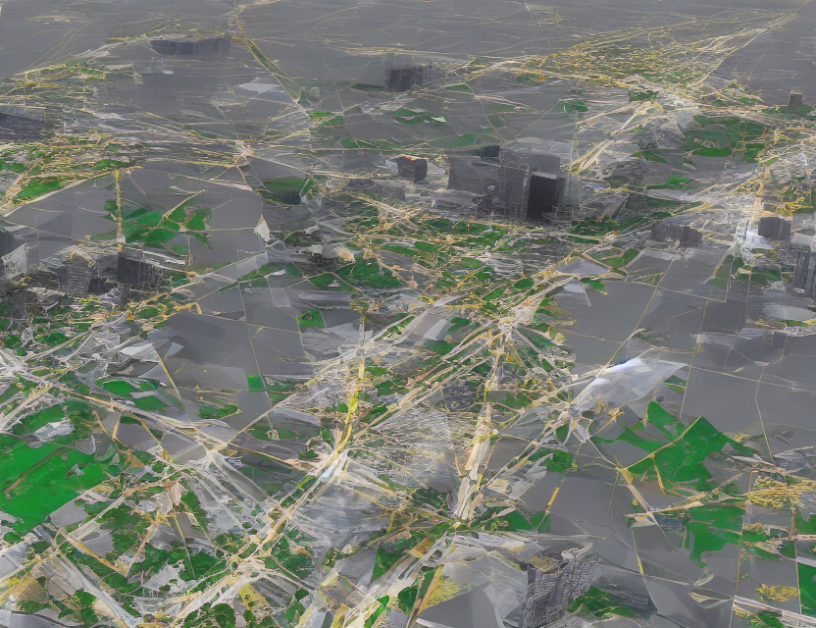