In the world of proteins, centrality measures are like maps that help us navigate through complex networks. However, these maps can be inaccurate if not normalized properly. In this article, we will delve into the importance of normalizing protein centrality scores in refined networks to ensure accurate evaluations.
Imagine a city with many interconnected roads. If you were to create a map without normalizing the distances between each road, you would end up with an inaccurate representation of the city’s layout. Similarly, in protein networks, centrality measures are calculated based on various properties such as degree, closeness, and betweenness. However, these measures can be affected by the size and complexity of the network, leading to inaccurate evaluations.
To overcome this challenge, researchers use normalization techniques to scale down or up the centrality values in each refined network. By adding a small deviation of 0.001 to the normalization process, they ensure that the centrality values in each network are on the same scale, making it easier to compare proteins across different networks.
In summary, normalizing protein centrality scores is crucial for comprehensive evaluations. It helps us navigate through complex networks with accuracy and makes sure we’re comparing apples to apples. By using everyday language and engaging analogies, we can demystify complex concepts and make the article accessible to a wide audience.
Molecular Networks, Quantitative Biology
Machine Learning Algorithms for Medical Image Analysis
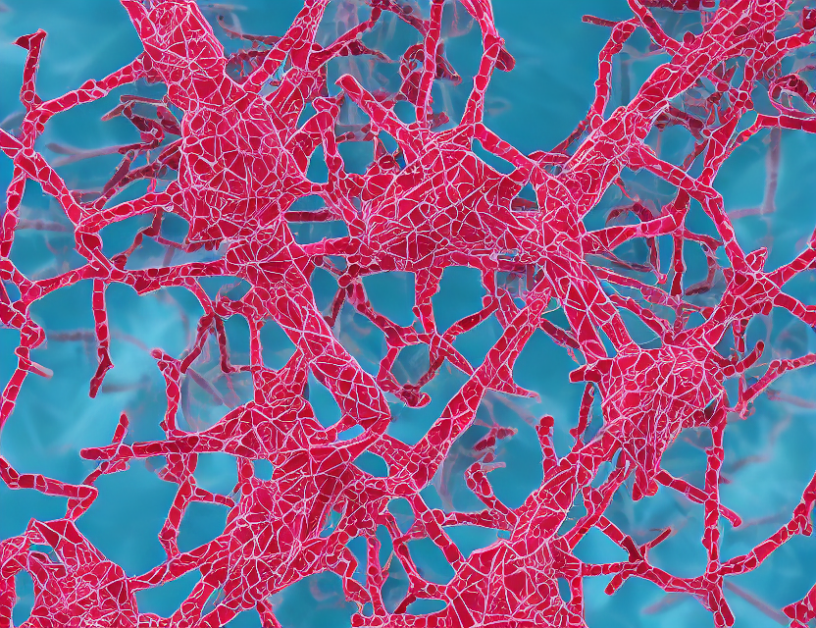