In this article, we delve into the realm of Bayesian deep learning to tackle the complexities of quantum many-body systems. Quantum mechanics, the branch of physics that governs the behavior of matter at the atomic and subatomic level, is inherently probabilistic in nature. This means that instead of deterministically predicting the outcome of a measurement, we assign probabilities to different outcomes based on the state of the system. Bayesian deep learning, which combines the power of deep neural networks with Bayesian inference, provides a framework for modeling and predicting quantum many-body systems.
To begin, let’s break down the term "Bayesian deep learning". Bayesian inference is a method of updating probabilities based on new data or information. In the context of deep learning, this means we can use Bayesian methods to update our predictions about the state of a quantum system as we receive more measurements. Deep neural networks are powerful models capable of learning complex patterns in large datasets. By combining these two approaches, we can create a framework for modeling quantum many-body systems that is both flexible and accurate.
Now, let’s dive into the specifics of how Bayesian deep learning works for quantum many-body systems. The first step is to define a quantum state, which encodes all the information about the quantum system we want to study. This state can be represented by a wave function, which is a mathematical object that describes the probability of finding the system in different states. Next, we need to define an observation or measurement that can be performed on the system. This could be something like detecting the position of a particle or measuring its momentum. Once we have defined these quantities, we can use Bayesian inference to update our probabilities for the quantum state based on new measurements.
One of the key advantages of Bayesian deep learning is that it allows us to model complex quantum systems without assuming any specific form for the wave function. This makes it particularly useful for studying systems where traditional methods fail, such as those with many degrees of freedom or those subject to strong interactions.
Now, let’s talk about some of the challenges associated with Bayesian deep learning for quantum many-body systems. One of the biggest hurdles is the computational cost of performing Bayesian inference. This can be computationally expensive, especially when dealing with large datasets or complex models. Another challenge is the difficulty in defining meaningful observables or measurements for the system under study. This can lead to difficulties in defining the prior probabilities or the likelihood function, which are essential components of Bayesian inference.
To overcome these challenges, researchers have developed various techniques and strategies. For instance, they may use approximations to reduce the computational cost or employ methods such as variational inference to improve the efficiency of the algorithm. They may also develop new observables or measurements that better capture the relevant features of the quantum system.
In conclusion, Bayesian deep learning offers a powerful approach for modeling and predicting complex quantum many-body systems. By combining the flexibility of deep neural networks with the probabilistic nature of Bayesian inference, we can create accurate models that capture the underlying physics of these systems. While there are challenges associated with this method, researchers continue to develop new techniques and strategies to overcome them and improve our understanding of quantum mechanics.
Bayesian Deep Learning and Probabilistic Perspective of Generalization
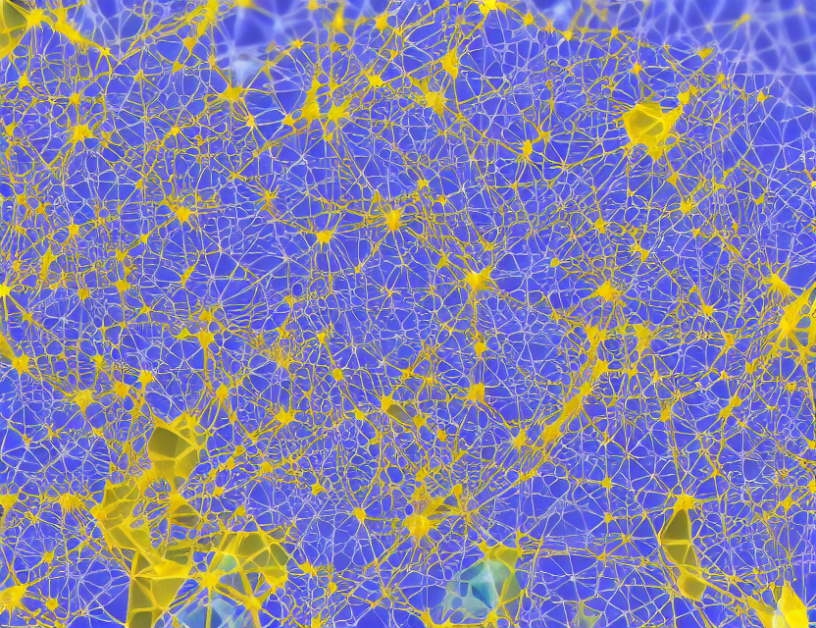