In this study, researchers compared the performance of three machine learning models – GNN, LSTM, and MLP – in predicting PM2.5 concentrations using data from prescribed fires. They found that the GNN model resulted in the lowest mean absolute error (MAE) and root mean squared error (RMSE) among the three models. The study also investigated the impact of fire radiative power (FRP) aggregation on PM2.5 predictions, finding that using FRP values within a certain radius of the PM2.5 monitor site improved prediction accuracy.
The researchers used data from CalFire’s prescribed burns dataset and split the data into training and testing sets. They used the GNN model to predict PM2.5 concentrations for both the training and testing sets and compared the results to the actual values. The study found that the GNN model had the lowest MAE and RMSE among the three models, with an average error of 150.5 µg/m3 for very unhealthy and hazardous PM2.5 levels.
The researchers also explored the impact of FRP aggregation on PM2.5 predictions. They found that using FRP values within a certain radius of the PM2.5 monitor site improved prediction accuracy, especially at larger distances. The study suggested that incorporating FRP values into the model could help improve the accuracy of PM2.5 predictions for prescribed fires.
Overall, the study demonstrated the effectiveness of GNN models in predicting PM2.5 concentrations and highlighted the importance of considering FRP aggregation when using machine learning models to predict air quality. The findings of this study have implications for environmental management and policy-making, particularly in areas where prescribed fires are used to mitigate wildfire risk.
In simple terms, the study compared three different machine learning models to see which one was best at predicting PM2.5 concentrations using data from prescribed fires. The researchers found that a new type of model called GNN performed the best and suggested that including information about the strength of nearby fires (called "FRP") can help make more accurate predictions. This study could help inform decisions about how to manage forests to reduce wildfire risk and protect air quality.
Atmospheric and Oceanic Physics, Physics
Fire Management and Predictive Analytics in California
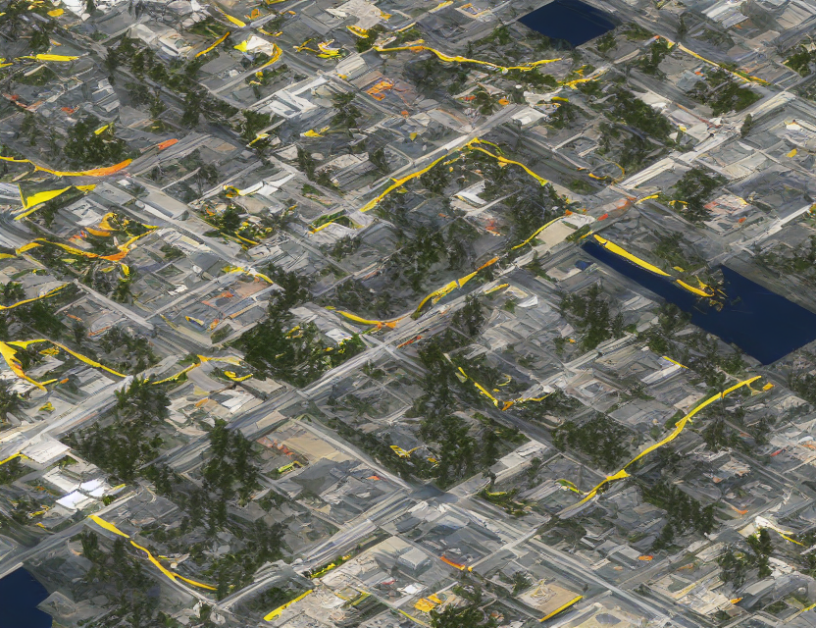