In this article, we explore the use of deep learning models for audio-visual speech recognition, with a focus on the impact of different decoders and supervised pre-trained models. We investigate how these factors affect the performance of audio-visual speech recognition systems and demonstrate the importance of carefully selecting these components.
Decoders: The authors compare the performance of different decoders, including BLSTM, Conformer, cross-attention, and Transformer. They find that Transformer yields the best results, with a DER reduction of 9.54%.
Supervised Pre-Trained Models: The authors examine the contribution of the supervised pre-trained model to the speaker encoder and find that it contributes more to the speaker encoder, which is related to the model’s training objective. This suggests that careful selection of the supervised pre-trained model is essential for optimal performance in audio-visual speech recognition tasks.
Conclusion: The study demonstrates the importance of carefully selecting the decoder and supervised pre-trained model for audio-visual speech recognition tasks. By using Transformer as the decoder and fine-tuning the supervised pre-trained model, the authors were able to achieve the best results, with a DER reduction of 9.54%. These findings have important implications for the development of audio-visual speech recognition systems, highlighting the need to carefully consider these factors in order to achieve optimal performance.
Audio and Speech Processing, Electrical Engineering and Systems Science
Improved Audio-Visual Speech Distortion Detection with Deep Learning Models
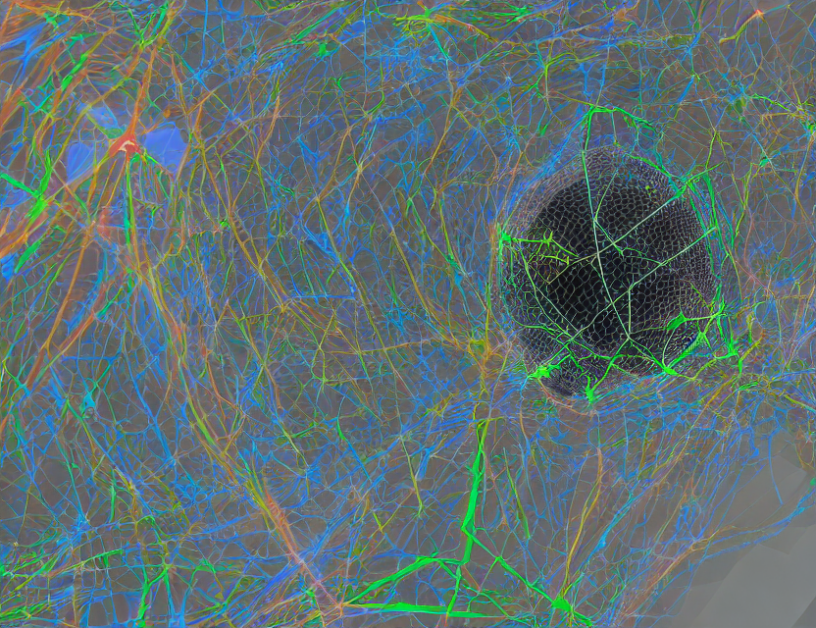