In this paper, the authors aim to simplify the evaluation of video compression algorithms by considering only the average PSNR (Peak Signal-to-Noise Ratio) gain instead of the complex architecture of video codecs. They propose using cosine similarity as an alternative metric that takes into account the context given by the average signal level, which is the achieved PSNR gains’ average. This approach can provide a more accurate measure of similarity between signals compared to Pearson’s correlation, which discards the average signal level when calculating the similarity.
The authors argue that using cosine similarity can help avoid some of the drawbacks of Pearson’s correlation, such as ignoring the context given by the average signal level. They also provide examples of how this approach can be applied in practice and demonstrate its effectiveness through simulations.
To understand this concept better, imagine you have two different types of fruit – apples and bananas. Apples are typically larger than bananas and have a thicker skin, while bananas are smaller and have a smoother skin. Now imagine you want to compare the similarity between these two types of fruit based on their size and skin thickness. Pearson’s correlation might tell you that apples and bananas are similar because they both have a certain amount of thickness in their skin, but it wouldn’t take into account the fact that one is much larger than the other. Cosine similarity, on the other hand, would look at the overall context of both fruits, including their size and skin thickness, to provide a more accurate measure of their similarity.
In summary, the authors propose using cosine similarity as an alternative metric for evaluating video compression algorithms, which takes into account the context given by the average signal level. This approach can provide a more accurate measure of similarity between signals compared to Pearson’s correlation and can help avoid some of its drawbacks.
Electrical Engineering and Systems Science, Image and Video Processing
In-Loop Reshaping: A Key to Improving Image Quality and Efficiency
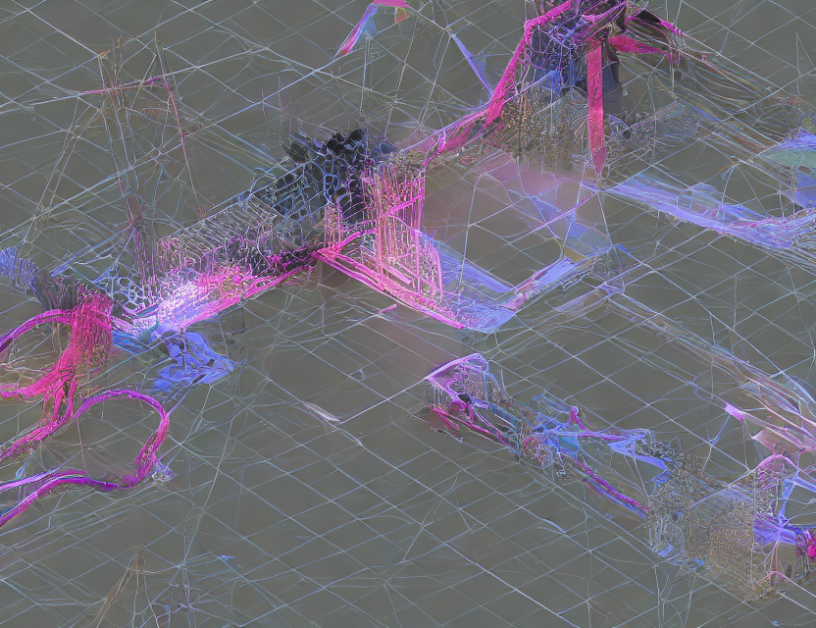