Safety-Enhanced Self-Learning for Optimal Power Converter Control
In this article, we propose a novel approach to optimize power converter control in microgrids, combining model predictive control (MPC) and reinforcement learning (RL). MPC is a powerful control technique that solves an optimization problem at each time step to determine the optimal control action, while RL learns from experiences to improve its policy over time. Our approach integrates these two techniques to ensure both robust and fast operation of ac microgrids while ensuring safety.
The challenge with traditional RL is that it can take a long time for the agent to converge to the optimal policy, especially in the initial stages where random exploration is involved. This makes it difficult to apply RL in practical scenarios, such as power electronics. To address this issue, we adopt the safe RL paradigm, which prioritizes safety over other objectives to ensure the learning process is safe and robust.
Our proposed approach uses a finite control-set model predictive control (FCS-MPC) framework to handle the optimization problem, while the RL agent learns from experiences to improve its policy. The FCS-MPC ensures that the power converter operates within a safe region, avoiding potential safety hazards. We evaluate our approach through simulations and demonstrate its effectiveness in optimizing power converter control for fast and robust operation of ac microgrids while ensuring safety.
In summary, this article proposes a novel approach to optimize power converter control in microgrids using MPC and RL, ensuring both robust and fast operation while prioritizing safety. Our proposed approach overcomes the limitations of traditional RL by adopting the safe RL paradigm, making it more practical for real-world applications.
Electrical Engineering and Systems Science, Systems and Control
Reinforcement Learning-Based Safety Policy for Robust Power Converter Control
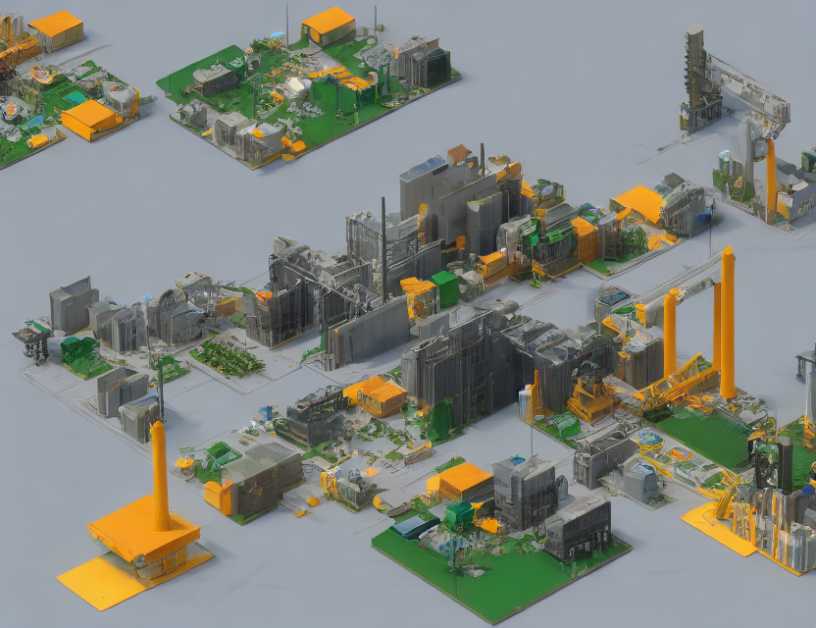