In this paper, researchers explore the use of masked autoencoders (MAEs) for unsupervised alignment of brain MRI scans. MAEs are a type of deep learning model that can learn to reconstruct images from incomplete or corrupted inputs. The authors propose using MAEs as a scalable vision learner for UAD, as they have shown potential in capturing the underlying healthy distribution of brain MRIs.
The limitations of traditional autoencoders (AEs) are addressed by adding skip connections with dropout, utilizing multi-scale features, or employing feature activation maps. Online outlier removal is also proposed for MAEs to improve their performance. Moreover, variational autoencoders (VAEs) have been investigated as an alternative approach to UAD, focusing on enhancing the used context in 2D and 3D.
To better understand the concept of masked autoencoders, imagine a game of "Guess the Image." Just like how we cover parts of an image with stickers to make it harder for someone to guess the original image, the masked autoencoder covers parts of the brain MRI with noise or blur. The model then tries to reconstruct the original image from the obscured areas, similar to how we try to guess the original image from the covered parts.
The authors demonstrate that MAEs can learn to reconstruct high-quality images even when a significant portion of the data is corrupted or missing. This makes them a promising approach for UAD in brain MRI, where the scans are often noisy or distorted due to various factors such as patient movement or scan artifacts.
The paper also explores the use of skip connections and multi-scale features to improve the performance of MAEs. Skip connections allow the model to learn more complex representations by adding information from higher levels of the network, while multi-scale features enable it to capture both local and global patterns in the data. These techniques help the model to better reconstruct the original image from the corrupted input.
In summary, masked autoencoders offer a promising approach for unsupervised alignment of brain MRIs, by learning to reconstruct high-quality images from noisy or distorted inputs. The authors propose several techniques to improve the performance of MAEs, including skip connections and multi-scale features. These advancements can help pave the way for more accurate and efficient UAD methods in brain MRI.
Electrical Engineering and Systems Science, Image and Video Processing
Scalable Vision Learners: A Comparative Study of Masked Autoencoders and Deep Diffusion Probabilistic Models for Image Reconstruction
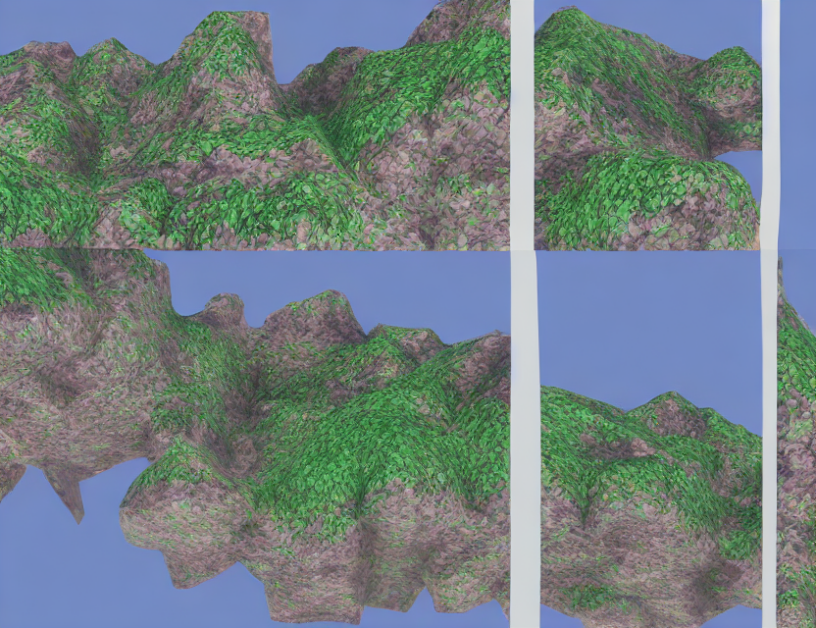