In this article, we explore the crucial process of data preprocessing and augmentation in materials science image analysis. By leveraging the power of machine learning algorithms, we can train a trustworthy classifier to accurately distinguish between different materials based on their atomic resolution STM images. However, before doing so, we need to ensure that our data is in good shape for the classifier to learn from it.
To begin with, all authors contributed to the conception, design, and implementation of the study. Material synthesis was performed by Luke Holtzman, while material preparation was carried out by Stephanie D. Lough. STM data collection of WSe2 and MoSe2 were performed by Darian Smalley and Madisen Holbrook, respectively. All deep learning and data analysis were performed by Darian Smalley, who also wrote the first draft of the manuscript.
Next, we discuss the importance of data preprocessing in materials science image analysis. Images can come in various formats, such as atomic resolution STM images, which are essential for identifying the atomic structure of materials. However, these images can be challenging to work with due to factors like noise and artifacts. To overcome these limitations, we apply a series of data preparation techniques, including plane correction, smoothing, and contrast enhancement. These operations help improve the clarity of features in the images and make them more suitable for machine learning analysis.
After preprocessing the data, we turn our attention to data augmentation. By randomly cropping regions from labeled STM images, we create new training data that can be used to train a classifier. This process helps improve the model’s performance by exposing it to a wider range of images and reducing overfitting. We also split the data into three groups: training, validation, and test sets, which are used to optimize hyperparameters and evaluate the model’s accuracy.
Finally, we present the results of our experiments, demonstrating that a U-Net ensemble can accurately classify materials based on their atomic resolution STM images. The ensemble achieves an F1 score of 0.69 and 0.63 for the peak and trough classes, respectively, with a high true positive rate and low false positive rate.
In conclusion, data preprocessing and augmentation are crucial steps in materials science image analysis. By carefully preparing and expanding the training data, we can train a reliable classifier to identify materials based on their atomic structure. These techniques help ensure that our model is robust enough to generalize well to new images and make accurate predictions.
Data Preprocessing and Augmentation: A Key to Unlocking Robust Feature Disentanglement in Imaging Data
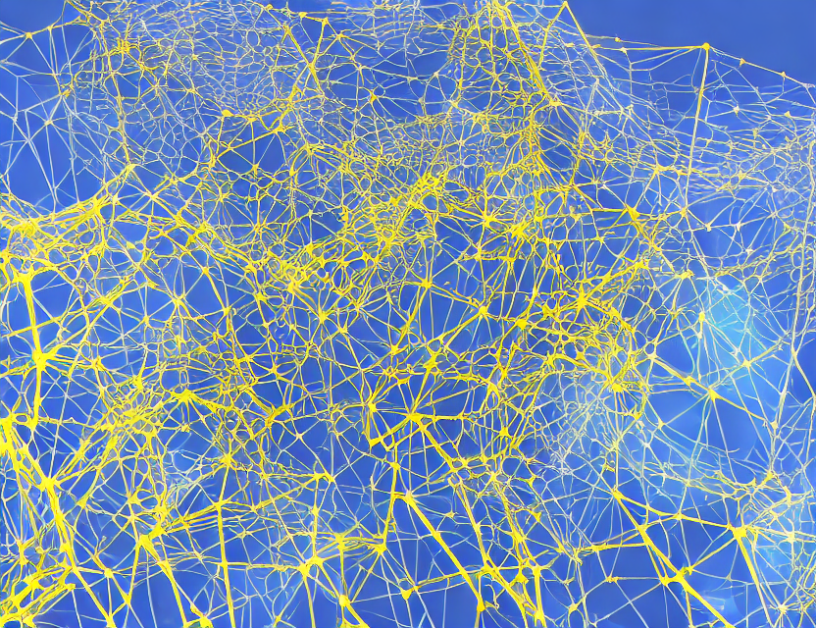