In this article, we explore the application of machine learning techniques to solve nonlinear dynamical systems. These systems are complex and difficult to analyze using traditional mathematical methods. The authors propose a novel approach called SciANN, which combines the power of neural networks with the insights of dynamical systems theory.
SciANN works by fitting a neural network to the data generated by the nonlinear system over time. The network is trained to predict the solution of the system at different points in time. This approach allows for the identification of the underlying mechanisms driving the behavior of the system, which can then be used to select the most relevant mechanisms and simplify the model.
The authors demonstrate the effectiveness of SciANN through several examples, including a van der Pol oscillator and a Lorenz system. In each case, they show how the algorithm can successfully identify the underlying dynamics and reduce the complexity of the model. They also discuss some of the challenges associated with this approach, such as the need for high-quality data and the potential for overfitting.
One of the key advantages of SciANN is its ability to handle complex systems with many interacting mechanisms. Traditional methods often struggle with these types of systems, as they can become computationally intractable. However, by using machine learning techniques, SciANN can efficiently identify the most important mechanisms and simplify the model without losing accuracy.
In conclusion, SciANN represents a significant breakthrough in the field of nonlinear dynamical systems analysis. Its ability to handle complex systems and provide accurate predictions makes it an invaluable tool for researchers and practitioners alike. As data collection and computational power continue to improve, we can expect to see even more advanced applications of this technology in the future.
Mathematics, Numerical Analysis
Learning Reduced Models for Nonlinear Dynamics via Selection
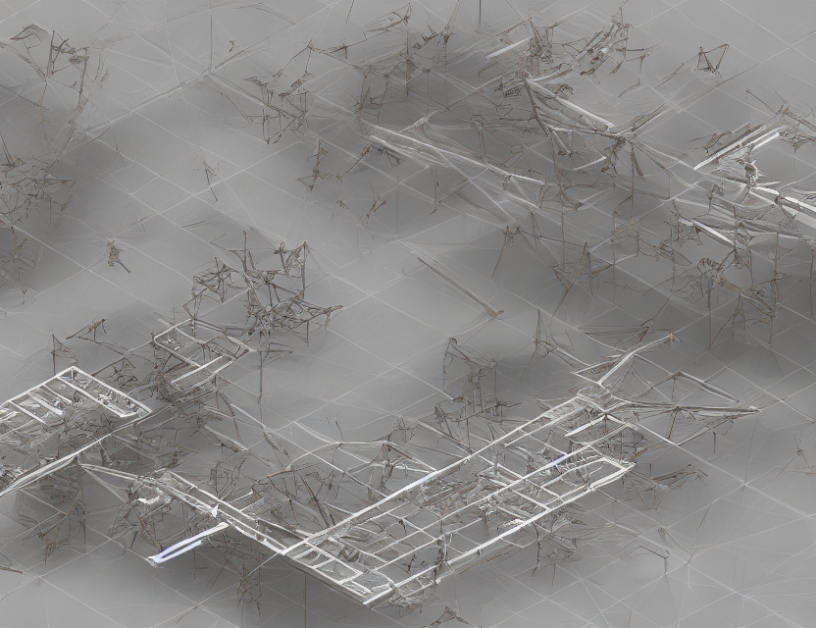