In this article, the authors propose using deep learning to design more efficient physical layer solutions in wireless communications. They argue that traditional approaches to physical layer design are limited by their reliance on mathematical models and lack of adaptability to changing channel conditions. By contrast, deep learning algorithms can learn complex patterns in the communication channel and adapt the physical layer accordingly, leading to improved efficiency and performance.
The authors describe two main approaches to using deep learning for physical layer design: (1) end-to-end learning, where the entire physical layer is learned from data, and (2) parameter sharing, where a shared neural network is used to learn both the transmit waveform and receiver algorithm. They show that these approaches can significantly improve the performance of wireless communication systems, especially in scenarios with highly dynamic channels.
To address challenges associated with end-to-end learning, such as the risk of overfitting or local minima, the authors introduce a new loss function called "dq" (θ), which penalizes closely spaced constellation points and encourages the algorithm to explore a more diverse set of solutions. They also propose using a hybrid approach that combines end-to-end learning with parameter sharing to leverage the strengths of both methods.
The authors demonstrate the effectiveness of their proposed approaches through simulations of a wireless communication system, showing that they can significantly improve the system’s performance compared to traditional methods. They also highlight the potential for further advancements in this area through the integration of additional techniques, such as transfer learning and meta-learning.
In summary, the article presents a promising approach to using deep learning for efficient physical layer solutions in wireless communications. By leveraging the adaptability and learning capabilities of neural networks, the proposed methods have the potential to significantly improve the performance and efficiency of wireless communication systems, especially in scenarios with highly dynamic channels.
Electrical Engineering and Systems Science, Signal Processing
Neural Network Transformation for Efficient Physical Layer Solutions
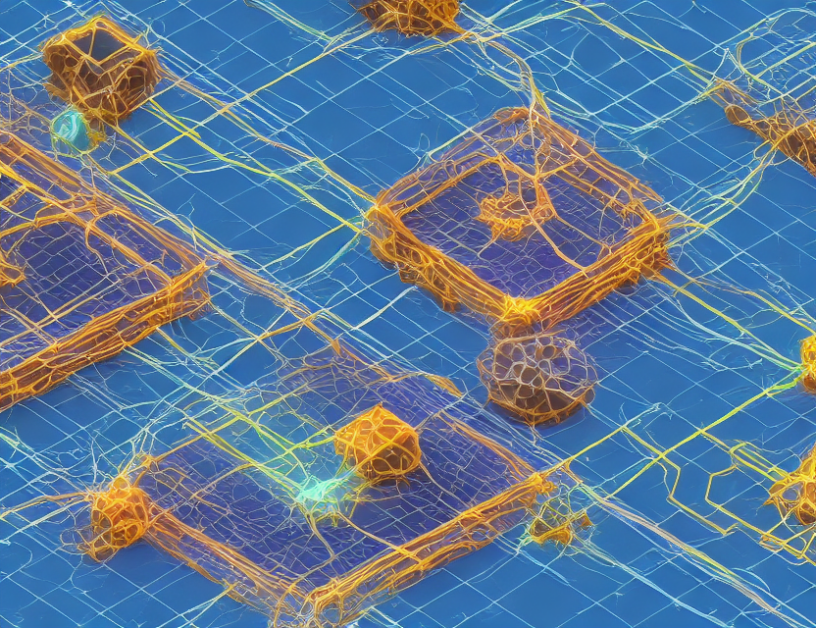