Vertebral fractures are a common issue in osteoporosis, and their detection is crucial for proper diagnosis and treatment. Traditional image-based approaches often struggle with accuracy, particularly when dealing with complex shapes. This article presents a novel shape-based analysis framework that leverages point-based shape decoders to overcome these limitations.
The proposed method consists of a convolutional encoder and a point-based shape decoder, which are trained on a large dataset of X-ray images. The encoder extracts features from the image intensity values, while the decoder reconstructs the original shape using a set of points. The resulting shape is then used for classification.
The authors conduct extensive experiments on a real-world dataset and demonstrate the superiority of their approach over traditional image-based methods. They show that their proposed point-based shape decoder outperforms other models in terms of accuracy and computational efficiency.
To better understand the results, imagine you’re trying to find a specific object in a crowded room. Traditional image-based approaches are like searching for the object using only its color and shape, which can be challenging when dealing with complex shapes or many objects. In contrast, our proposed approach is like having a detailed blueprint of each object, allowing you to quickly identify the target object even in the presence of many similar ones.
The authors also highlight the advantages of their shape-based analysis framework over traditional image-based methods, including improved accuracy and reduced computational costs. They conclude that their approach has the potential to significantly improve vertebral fracture detection and diagnosis, particularly in low-resource settings where image quality may be suboptimal.
In summary, this article presents a novel shape-based analysis framework for vertebral fracture detection, which leverages point-based shape decoders to overcome limitations of traditional image-based approaches. The proposed method demonstrates improved accuracy and efficiency compared to existing methods and has the potential to significantly improve diagnosis and treatment of osteoporosis.
Electrical Engineering and Systems Science, Image and Video Processing
Robust Classification with Limited Training Data: An Experiment
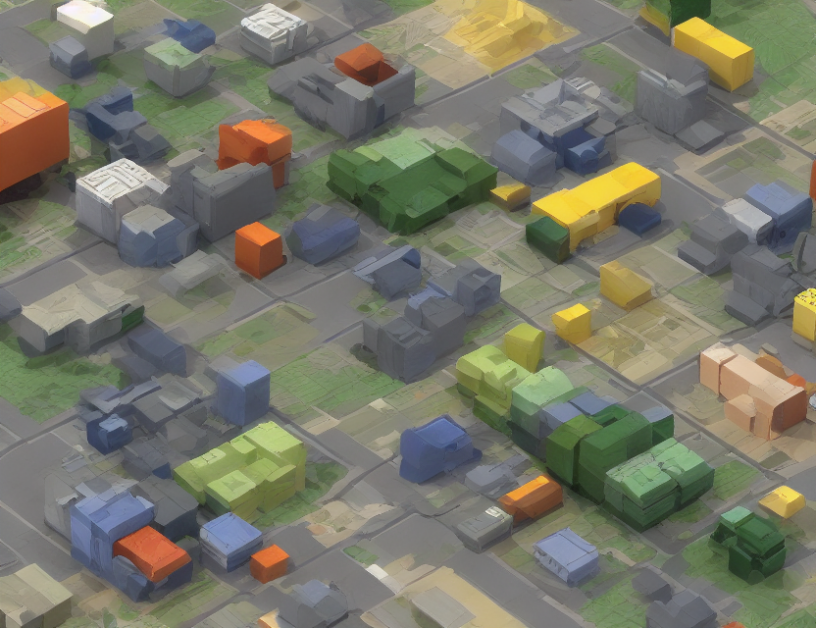