In this article, we delve into the realm of non-parametric regression, where traditional statistical methods often fall short. The authors introduce a new approach to constructing confidence intervals for regression coefficients in the presence of uncertainty, leveraging sequential testing and adaptation. By incrementally updating our understanding of the underlying distribution through iterative sampling, we can create more accurate prediction intervals that adapt to changing data distributions.
The key insight lies in the concept of "sequential inductive inference," which enables us to construct a confidence interval for the regression coefficient based on a small sample of data and then iteratively update this interval as new data points become available. This process mimics the idea of sequential testing, where we gradually refine our estimation of the parameter based on increasing evidence.
The authors present two main results: (1) an upper bound on the true coverage probability of the constructed intervals, and (2) a data-dependent upper bound on the true coverage probability when using Theorem 2 instead of the three-parameter PAC. These bounds provide a means to evaluate the accuracy of our confidence intervals and ensure they are reasonably close to the true quantiles.
To construct these intervals, we first estimate the regression coefficient using the Nadaraya-Watson estimator with a Gaussian kernel of bandwidth 1/500. We then compute the score function and construct a prediction interval for the regression coefficient based on the score function. This process is repeated as new data points become available, allowing us to iteratively update our confidence intervals and improve their accuracy.
The article provides several examples and simulations to demonstrate the effectiveness of this approach in various scenarios. By leveraging sequential testing and adaptation, we can create more robust and reliable confidence intervals for non-parametric regression, making it easier to make predictions and quantify uncertainty in complex systems.
In summary, this article introduces a powerful new tool for constructing confidence intervals in non-parametric regression, allowing us to adapt our estimates to changing data distributions and improve our accuracy over time. By leveraging sequential testing and adaptation, we can create more robust and reliable predictions, making it easier to navigate the complex world of machine learning and statistical analysis.
Sequential Inductive Prediction Intervals: Leveraging New Data for Improved Accuracy
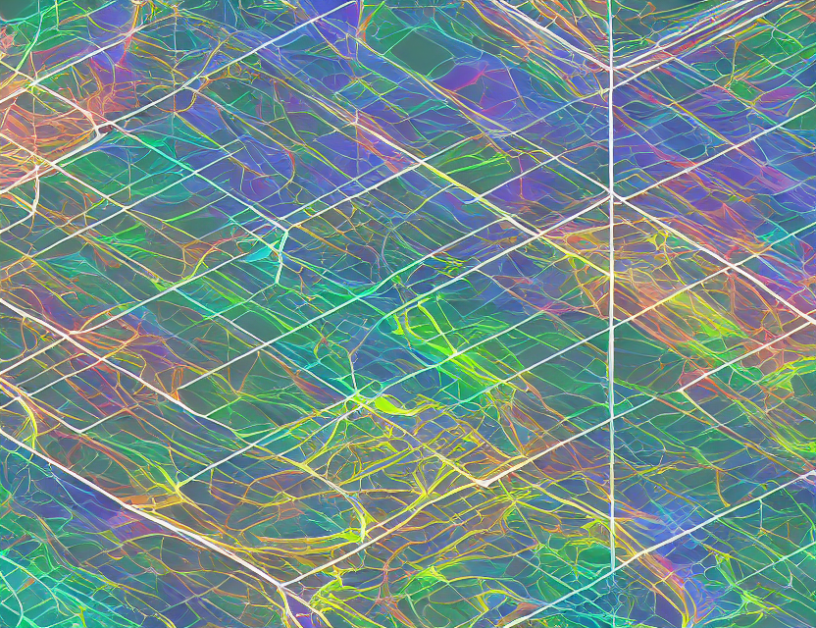