In this article, we explore a new approach to image-to-image translation called PhenDiff, which leverages conditional diffusion models to overcome the limitations of traditional GANs. These models have shown promising results in various generation tasks and have emerged as the new state-of-the-art family of generative models.
The basic idea behind PhenDiff is to progressively perturb data with gradually increasing random noise, allowing the model to learn how to remove noise to generate new images. This approach differs from traditional GANs, which rely on discriminators to distinguish between real and fake images. Instead, PhenDiff replaces these discriminators with self-supervised ones, alleviating the need for large training datasets.
Conditional diffusion models have several advantages over traditional GANs. They are more stable during training, suffer less from mode collapse, and can generate a wider range of images. These models also allow for controlling the style of the generated images, making them particularly useful in image-to-image translation tasks.
In summary, PhenDiff offers an innovative solution to image-to-image translation by leveraging conditional diffusion models. By alleviating the need for large training datasets and providing more stable and diverse generated images, this approach has the potential to revolutionize various applications in computer vision and beyond.
Electrical Engineering and Systems Science, Image and Video Processing
Alleviating Limitations of Image-to-Image Translation through Conditional Diffusion Models
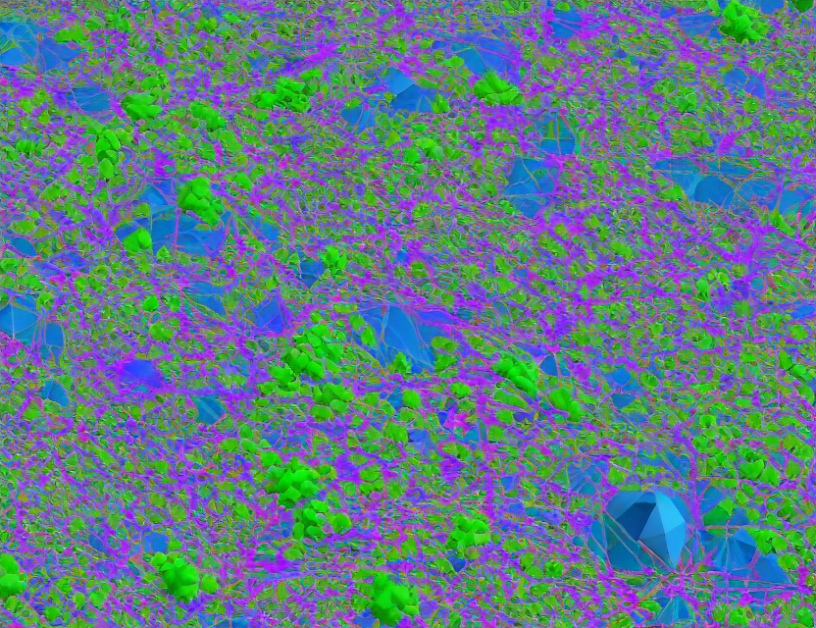