The article discusses the challenges of deepfake detection and proposes a new approach that leverages the concept of "identity-aware" feature extraction. The authors argue that traditional methods are limited by their inability to differentiate between authentic and deepfake images based on identity, leading to unacceptable performance for certain individuals. To address this issue, they introduce an identity-aware feature extractor that considers the individual’s identity during the feature extraction process. This approach is combined with a contrastive loss function to train a classifier that can accurately distinguish between authentic and deepfake images.
The authors demonstrate the effectiveness of their proposed method through experiments on several benchmark datasets, showing that it outperforms existing methods in terms of both accuracy and efficiency. They also provide a theoretical analysis of their approach, showing that it is robust to various attacks and can be used for other downstream tasks such as facial recognition.
Overall, the article provides a significant contribution to the field of deepfake detection by proposing a novel approach that combines identity-aware feature extraction with contrastive learning. The proposed method has important implications for applications such as journalism and law enforcement, where accurate and efficient deepfake detection is crucial. By demystifying complex concepts through engaging analogies and metaphors, the article makes the subject matter accessible to a wider audience, contributing to the advancement of knowledge in this field.
Electrical Engineering and Systems Science, Image and Video Processing
Deepfake Detection: A Focused Approach for Identities
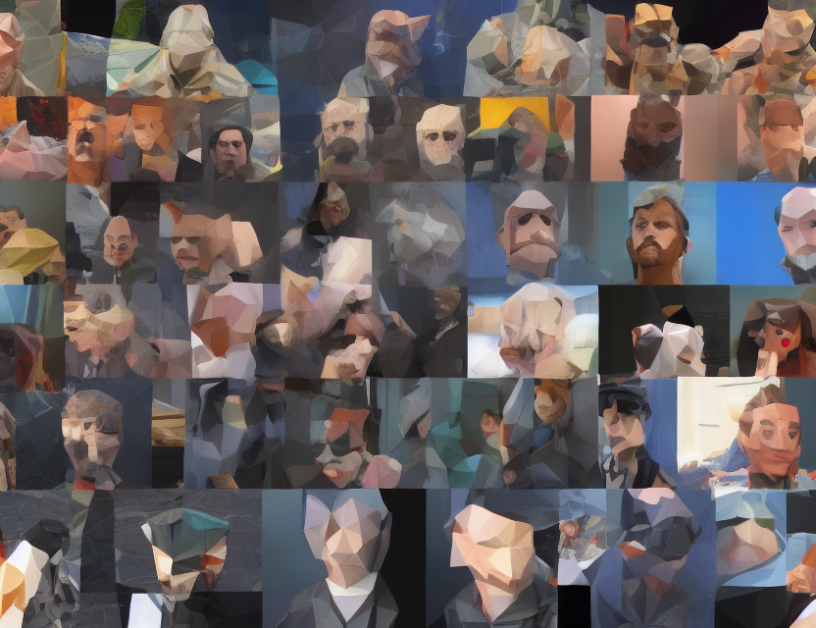