Imagine you’re a detective trying to estimate the amount of water in a patch of soil based on clues from an old, forgotten well. Traditional methods would involve digging up the well and measuring its depth, diameter, and water level manually. But what if you could use a high-tech tool to speed up the process and provide more accurate results? That’s where Bayesian model updating comes in!
Bayesian model updating is like playing a game of probability puzzles. Researchers start with a rough idea of the soil moisture level, then gather data from various sources (like GPR experiments or FDTD simulations), and use that information to update their estimate. It’s like solving a complex math problem, but instead of just getting one right answer, Bayesian model updating provides a range of possible solutions, each with its own probability of being correct.
This approach has many advantages over traditional methods. For starters, it allows researchers to incorporate prior knowledge about the soil moisture level, which can be especially useful when there’s limited data available. It also provides a probabilistic framework for model calibration and uncertainty quantification, giving a more accurate picture of how confident they are in their estimate. And if new data becomes available, Bayesian model updating can quickly update the model parameters using deterministic optimization methods like particle swarm optimization or genetic algorithms.
But wait, there’s more! Bayesian model updating is also more robust than traditional methods, providing a range of possible solutions instead of just one fixed answer. This means that not only can researchers estimate the soil moisture level more accurately, but they can also quantify the uncertainty associated with their estimates. Think of it like playing a game of probability poker – while you may have a good idea of the soil moisture level, there’s still some degree of chance involved, and Bayesian model updating helps to quantify that uncertainty.
In conclusion, Bayesian model updating is a powerful tool for estimating soil moisture levels. By using prior knowledge, incorporating new data, and providing a probabilistic framework for model calibration and uncertainty quantification, it offers a more accurate and robust approach than traditional methods. So next time you’re trying to solve a complex problem in the field of geophysics or materials science, remember – Bayesian model updating may be just the tool you need to unlock the secrets of the soil!
Electrical Engineering and Systems Science, Signal Processing
Deterministic vs Uncertainty-Aware Inversion of Soil Moisture and Surface Material
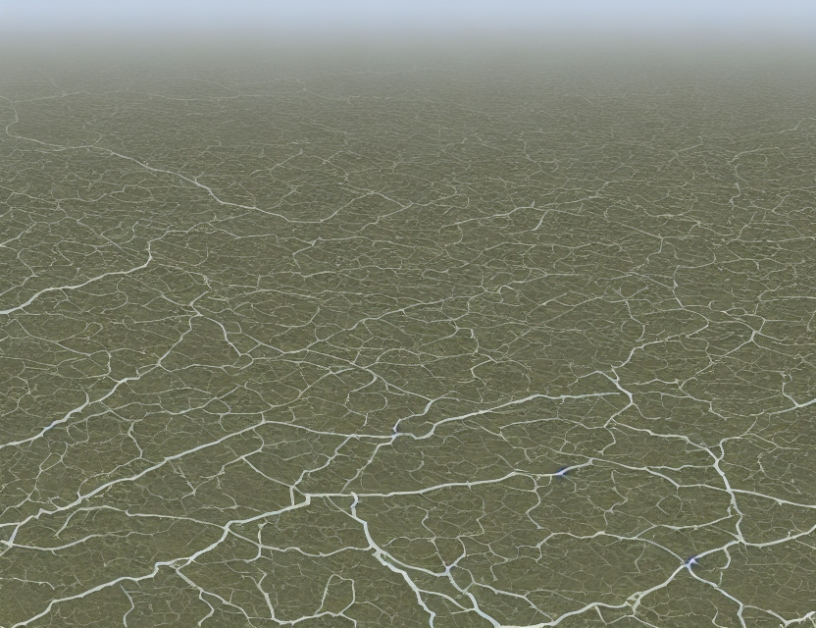