Scientific research relies on high-quality data to build accurate models and make meaningful discoveries. However, collecting and processing large datasets can be time-consuming and costly, limiting the scope of scientific inquiry. Traditional methods of data collection and analysis are often insufficient for complex systems, leading to a growing need for more sophisticated techniques.
In recent years, advances in machine learning have enabled the development of new methods for estimating and learning probability distributions. These approaches have shown great promise in modeling physical processes and generating new data and inferences. From density estimation to generative models, these techniques offer a powerful toolset for scientists to tackle complex problems.
One particularly promising approach is the use of deep neural networks to model probability distributions. These models are capable of learning complex patterns in large datasets, allowing researchers to make accurate predictions and gain new insights. By leveraging the power of these models, scientists can overcome the limitations of traditional data collection methods and unlock new discoveries.
However, it is important to recognize that these techniques are not without their challenges. The complexity of the models themselves can make them difficult to interpret and understand, and the sheer volume of data required for training can be overwhelming. Moreover, the computational resources needed to train these models can be prohibitively expensive, limiting access to those with the means to afford them.
Despite these challenges, the potential benefits of these techniques are substantial. By harnessing the power of machine learning and high-quality data, scientists can push the boundaries of what is possible in their field, leading to new discoveries and advancements that would otherwise be out of reach. As such, investment in these technologies is essential for unlocking the full potential of scientific research.
Computational Physics, Physics
Enhancing Scientific Data Quality through Diffusion-Based Methods
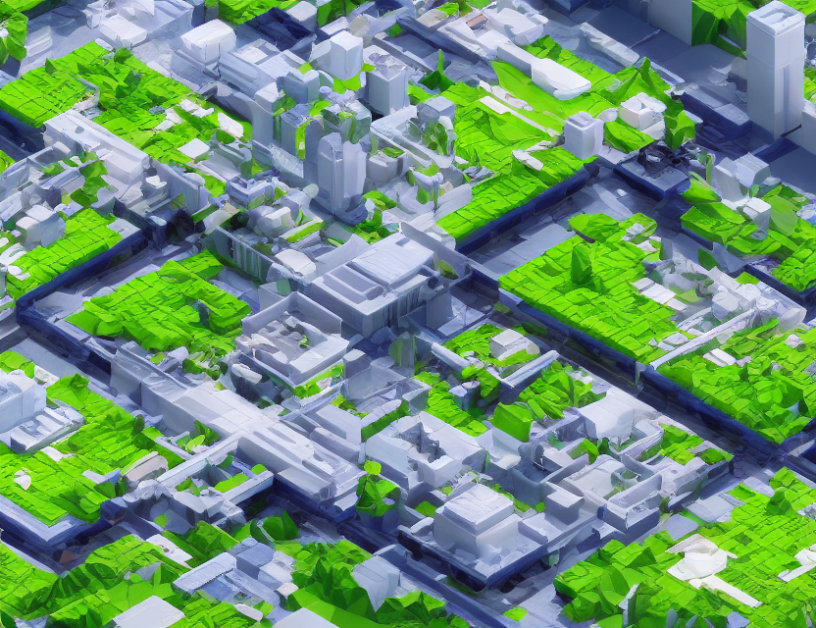