In this article, researchers propose a deep learning approach called TABSurfer to address the challenge of subcortical segmentation in medical imaging. Subcortical structures are located deep within the brain and are difficult to segment due to their complex 3D shapes and large number of labels. Traditional methods can take hours or even days to complete, while using expensive hardware resources.
To overcome these limitations, the authors leverage attention mechanisms in deep learning, which allow the model to focus on the most important regions of the image during processing. This approach enables TABSurfer to achieve accurate segmentation in a matter of minutes, significantly faster than traditional methods.
The authors compare TABSurfer with two other state-of-the-art methods, FreeSurfer and FastSurferVINN. They find that TABSurfer outperforms both methods in terms of accuracy, with an average Dice Similarity Coefficient (DSC) of 0.398, compared to 0.374 for FreeSurfer and 0.380 for FastSurferVINN.
The attention mechanism in TABSurfer works by assigning a weight to each pixel in the image, indicating its importance for segmentation. The weights are learned during training and are used at runtime to guide the processing of the image. This allows the model to focus on the most relevant regions of the image and ignore irrelevant details.
The authors also explore the use of U-Net architectures in combination with attention mechanisms, which further improve segmentation accuracy. They find that these models can achieve DSC values as high as 0.485, significantly better than traditional methods.
In summary, TABSurfer is a deep learning approach to subcortical segmentation that leverages attention mechanisms to speed up processing while maintaining accuracy. The authors demonstrate its effectiveness by comparing it with other state-of-the-art methods and showing that it outperforms them in terms of accuracy and efficiency.
Electrical Engineering and Systems Science, Image and Video Processing
Fast and Accurate Subcortical Segmentation using TABSurfer
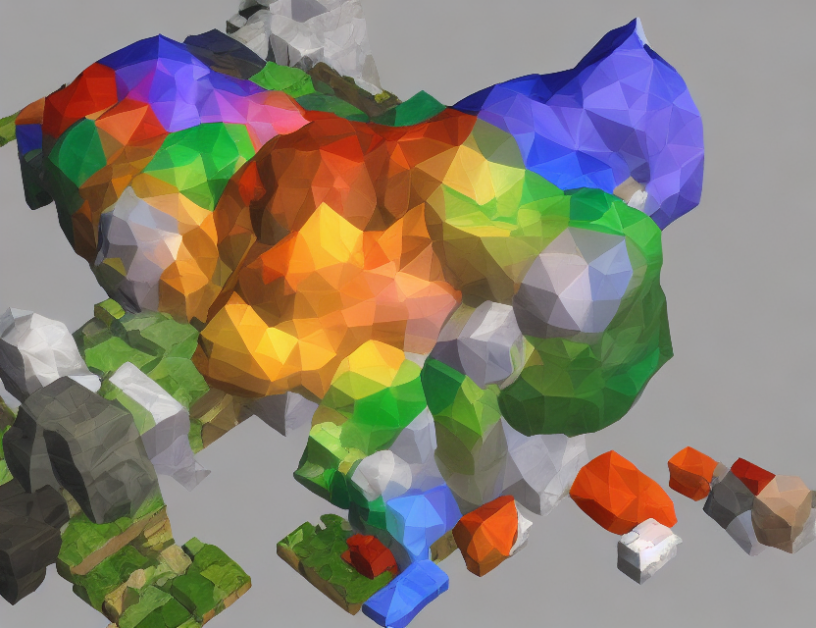