The OCTDL dataset is a collection of images captured using an optical coherence tomography (OCT) device, designed to support the development and evaluation of deep learning models for image-based medical analysis. The article provides an overview of the dataset’s composition, including its split into training, validation, and test sets, as well as details on data augmentation techniques used to enhance the dataset’s diversity.
The authors report that the dataset contains images from 12 eye diseases, with varying levels of complexity and difficulty to classify. They also highlight the balanced accuracy achieved across all categories, with AMD demonstrating the highest accuracy at 0.963, followed by DR at 0.932, and NO at 0.957.
To help readers understand the dataset’s complexity, the authors present confusion matrices for two popular deep learning models, ResNet50 and VGG16, which demonstrate how well these models perform in classifying different eye diseases based on the OCT images.
In summary, the OCTDL dataset is a valuable resource for researchers and developers working on image-based deep learning methods for medical analysis, particularly in the field of ophthalmology. The dataset’s balanced accuracy and diverse range of images make it an ideal platform for testing and refining new algorithms, with the potential to improve diagnosis and treatment of various eye diseases.
Electrical Engineering and Systems Science, Image and Video Processing
Image-Based Deep Learning Methods for Optical Coherence Tomography Dataset Analysis
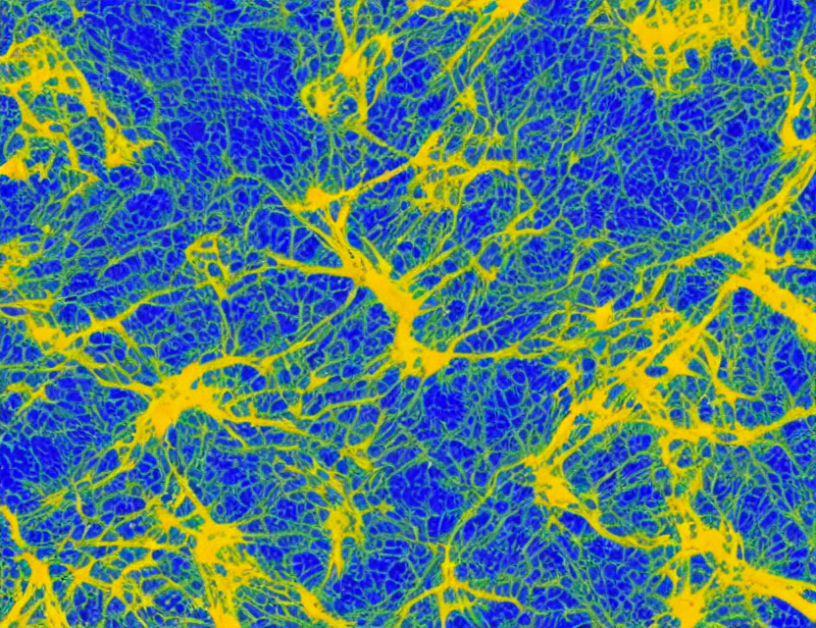