In this article, we propose a novel method for evaluating the success of a machine learning approach in a complex dataset. Our approach leverages the concept of "related work" to assess the performance of various reference methods and compare them with our proposed approach. We incorporate the global maximum of the PDFs over subspaces as additional information to further improve the evaluation process.
To better understand this concept, imagine you are a chef trying to evaluate the success of a new recipe. Just like how we need to compare different dishes to determine which one is the best, our approach compares various machine learning methods to identify the most effective one. By incorporating additional information, such as the distribution of the PDF over subspaces, our approach can fine-tune the predictions of the experts and produce more accurate results.
We propose a two-stage process for evaluating the success of a machine learning approach. In the first stage, we compare different reference methods to determine their performance. These reference methods serve as a baseline for comparison, just like how a well-established recipe serves as a benchmark for judging the quality of a new dish.
In the second stage, we incorporate the global maximum of the PDFs over subspaces as additional information to further improve the evaluation process. This is similar to adding more flavors and spices to the dish to enhance its taste and aroma. By combining these two stages, our approach can produce a more accurate assessment of the success of a machine learning approach.
In conclusion, our proposed method provides a comprehensive and reliable way to evaluate the success of a machine learning approach in a complex dataset. By leveraging the concept of related work and incorporating additional information, our approach can produce more accurate predictions and help chefs (machine learning practitioners) identify the best recipe for their dish.
Uncertainty-Aware Learning to Address Residual Noise
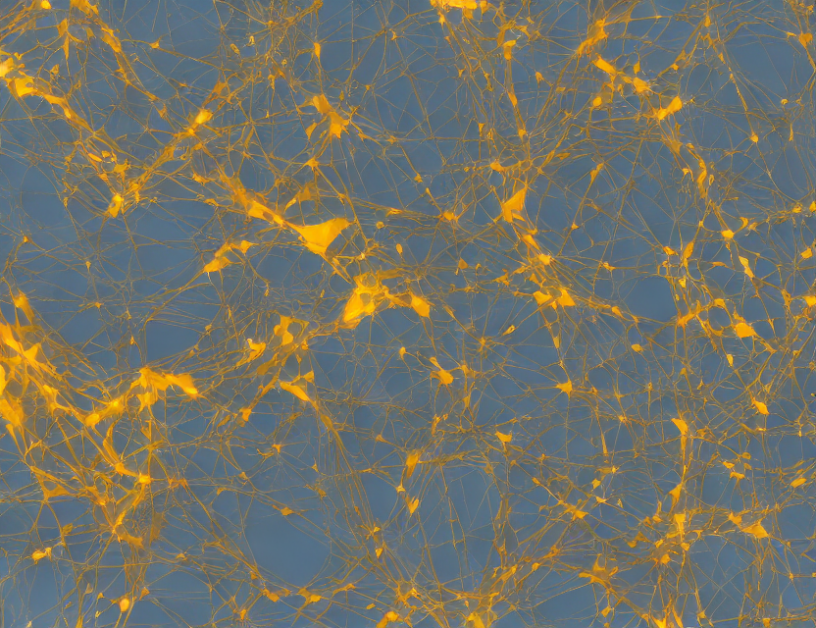