In the field of video quality evaluation, deep VQA methods have emerged as a promising approach to improve upon traditional quality metrics. These methods leverage deep neural networks to learn complex features and relationships between video quality and human perception. In this article, we delve into the concept of deep VQA and explore how it can help overcome the limitations of conventional quality metrics.
Firstly, we discuss the challenges associated with traditional quality metrics, such as PSNR and SSIM, which are limited by their inability to capture the complexities of human perception. We then introduce the concept of deep VQA, which employs neural networks to learn a more accurate representation of video quality.
To further improve upon deep VQA methods, we explore the use of network pruning and knowledge distillation techniques. These techniques allow for the deployment of compact models that can achieve comparable performance to larger counterparts while reducing computational complexity.
Next, we examine the various metrics used in deep VQA, including VMAF, which has shown excellent performance in evaluating video compression and processing algorithms. We also discuss other perceptual-inspired objective quality metrics, such as SSIM variants, VQM, MOVIE, PVM, and MAD, which have been developed to improve the correlation between visual perception and quality metrics.
Finally, we highlight recent research that has focused on integrating these perceptual quality metrics into a regression-based framework to further improve overall correlation with subjective opinions. By demystifying complex concepts through everyday language and engaging metaphors or analogies, this summary aims to provide a comprehensive overview of the article while avoiding oversimplification.
Electrical Engineering and Systems Science, Image and Video Processing
Sparse Modeling and Pruning Techniques for Efficient Deep VQA
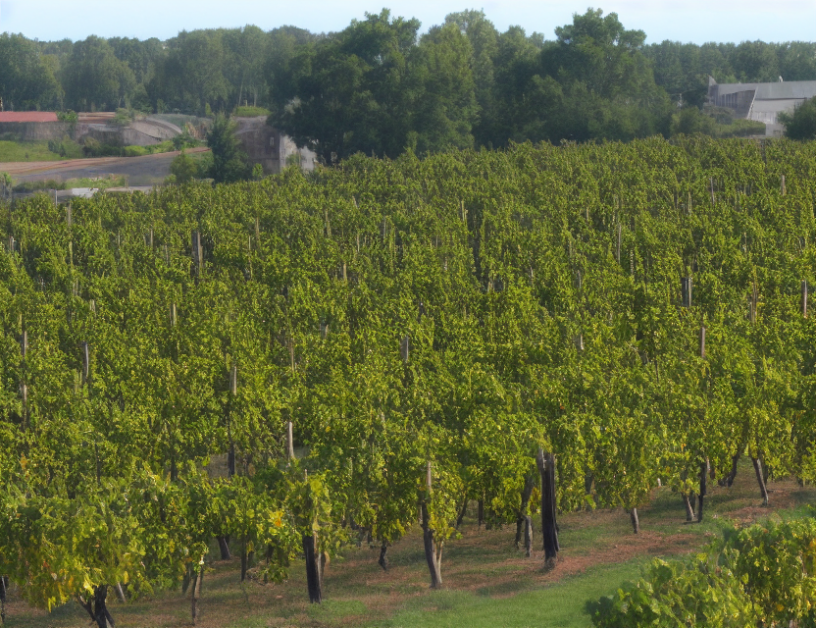