In this article, we explore the concept of code-switching in automatic speech recognition (ASR) and its importance in handling multilingual inputs. Code-switching refers to the ability of an ASR system to recognize and transition between different languages within a single sentence or utterance. This is a challenging task as it requires the system to understand the context and semantics of each language being used.
To address this challenge, researchers have developed foundation models such as XLS-R [3], Whisper [4], USM [5], and MMS [6]. These models are pre-trained from large corpora of audio data with numerous languages, enabling them to handle code-switching inputs in an encoder-decoder framework.
The article explains that the encoder part of the model is responsible for extracting the contextual information from the input speech, while the decoder generates the output transcription based on this context. The adaptation of the decoder to handle code-switching is achieved by adding a controllable adapter to the frozen backbone of the Whisper model [4]. This adapter allows the system to adapt to new languages in real-time, enabling it to recognize and switch between different languages seamlessly.
The article also provides examples of how code-switching can occur in everyday conversations, highlighting its prevalence in multilingual communities. It emphasizes that the ability to handle code-switching is crucial for ASR systems to achieve high accuracy and provide reliable transcriptions in real-world scenarios.
In summary, this article delves into the complexities of code-switching in ASR and explores innovative solutions to address this challenge. By leveraging cutting-edge technologies and techniques, researchers are paving the way for more accurate and efficient multilingual speech recognition systems.
Audio and Speech Processing, Electrical Engineering and Systems Science
Unlocking Hidden Talent: Speech Models for Zero-Shot Task Generalization
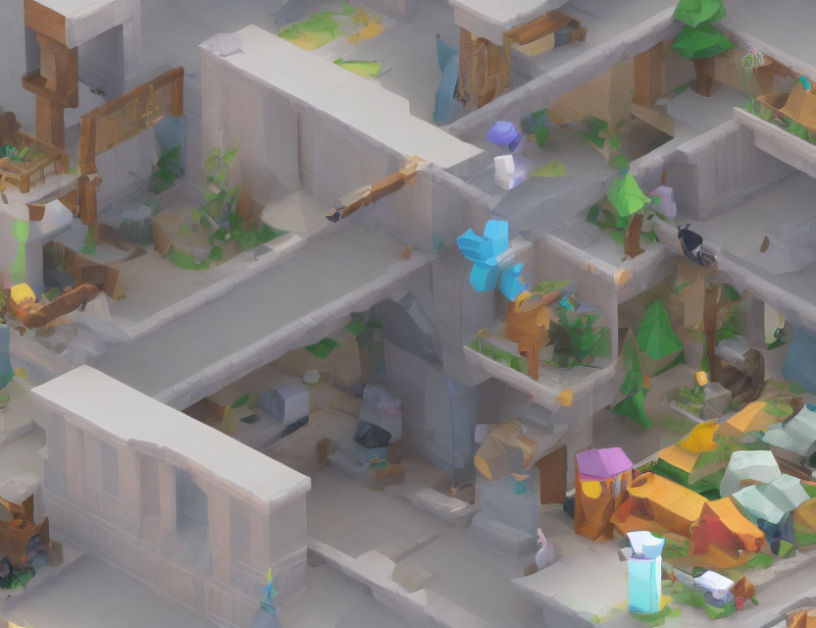