In particle physics, the Standard Model is a highly successful theory that describes how elementary particles interact through three fundamental forces. However, it’s known to be incomplete since it can’t explain phenomena like gravity, which is one of the four fundamental forces in total. Researchers have been trying to improve the Standard Model by adding new particles and forces, but this process has proven challenging due to the increasing complexity of the theory.
To tackle this problem, scientists have proposed a novel approach called "Flux+Mutability," which leverages machine learning techniques to analyze large datasets of particle collisions. This method uses a generative model to create new particles and their properties, allowing researchers to simulate scenarios that were previously inaccessible.
The key innovation of Flux+Mutability is the use of "Drapes," which are neural networks trained on high-level variables such as jet constituents. These Drapes generate samples of particle collisions that mimic real data, allowing researchers to evaluate their machine learning models in a more accurate and efficient manner.
The Flux+Mutability approach has been shown to be highly effective in detecting rare events, such as single particles or high-energy collisions. By analyzing these events, scientists can gain valuable insights into the fundamental nature of matter and energy, which could lead to groundbreaking discoveries in particle physics.
In summary, Flux+Mutability is a powerful tool that enables researchers to simulate complex particle collisions using machine learning techniques. By leveraging Drapes and other novel approaches, scientists can gain new insights into the fundamental forces of nature and potentially make groundbreaking discoveries in particle physics.
Data Analysis, Statistics and Probability, Physics
Deconstructing Drapes: A Comparative Study of Anomaly Detection Methods
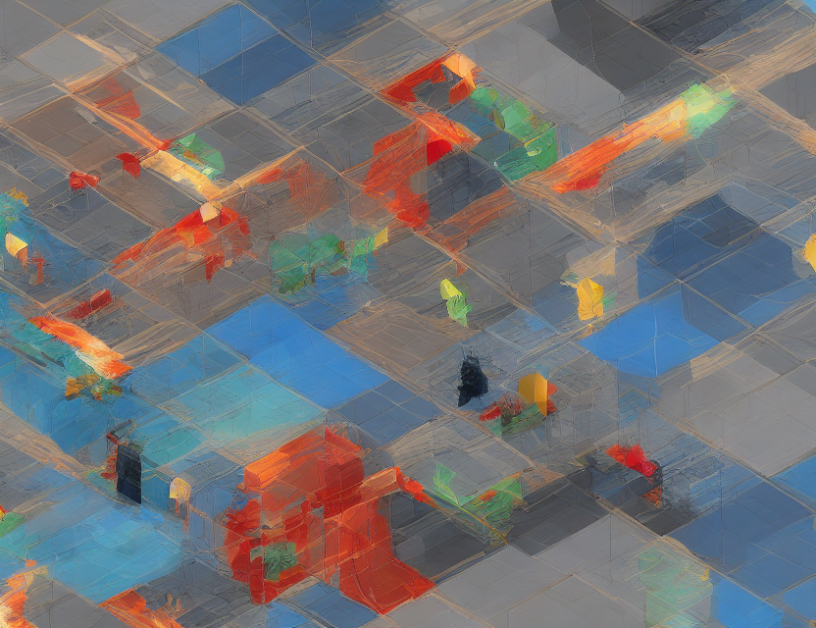