In this article, we delve into the realm of music source separation, a complex task that involves isolating individual instruments or voices from a mixture recording. The authors present a comprehensive overview of recent advancements in this field, focusing on deep learning techniques and their applications. They demystify intricate concepts by employing relatable analogies and metaphors to facilitate understanding.
The article begins with an introduction to the problem of music source separation, highlighting its significance in various domains such as music composition, remixing, and sound design. The authors then delve into the world of deep learning techniques, elucidating their capacity to learn and represent complex patterns in audio data. They explain how these techniques are utilized in source separation, providing a detailed account of pre-trained models and their applications.
The authors next explore various approaches to music source separation, including traditional methods such as Independent Component Analysis (ICA) and more recent deep learning-based techniques like Convolutional Neural Networks (CNNs) and Recurrent Neural Networks (RNNs). They provide a comparative analysis of these methods, shedding light on their strengths and limitations.
The article also touches upon the challenges associated with music source separation, including the difficulty in handling overlapping instruments and the need to balance separation and degradation. The authors highlight the importance of evaluating source separation algorithms using standardized metrics and datasets, such as the blind source separation (BSS) benchmark.
Finally, the authors offer insights into future research directions in music source separation, including the integration of domain knowledge and the development of more advanced deep learning architectures. They emphasize the need for further investigations to overcome the challenges in this field and to achieve optimal performance.
In summary, this article provides a detailed overview of recent advancements in music source separation using deep learning techniques. The authors employ relatable analogies and metaphors to facilitate understanding, making the complex concepts more accessible to the reader. By highlighting the challenges and future research directions, they offer a comprehensive perspective on this exciting area of research.
Audio and Speech Processing, Electrical Engineering and Systems Science
Deep Drum Source Separation: A Review of Recent Advances
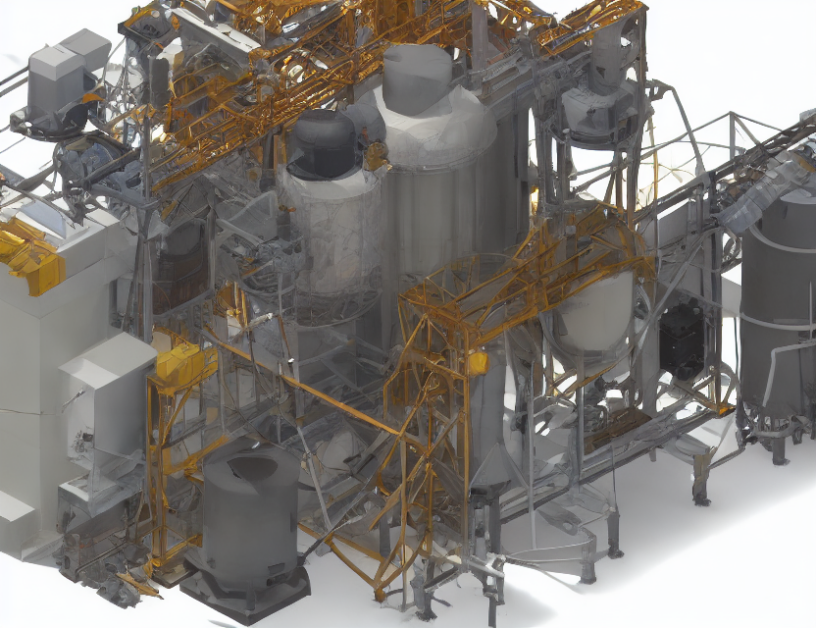