Decoding Speech Attention with Deep Neural Networks
In this study, researchers aimed to develop a novel approach for decoding auditory attention using deep neural networks. They trained their model on a dataset of continuous speech and achieved an accuracy rate of 95%. The team explored the impact of segment length on decoding accuracy and found that longer segments led to improved performance.
The researchers used three different approaches to enhance the accuracy of their system: fine-tuning individual listeners’ decoders, ensembling a population of distinct decoders by averaging over their predictions, and investigating generalization capabilities across different speech and listening conditions. They discovered that ensembling decoders yielded the best results, with an average increase in accuracy of 10%.
The study demonstrates the potential of deep neural networks for auditory attention decoding, a critical aspect of human communication. The researchers’ approach could pave the way for more accurate speech-to-speech translation and other applications that rely on understanding auditory attention.
By harnessing the power of deep learning, this study demonstrates how machine learning algorithms can be used to improve our ability to understand and interpret complex sounds like speech. It’s like having a superpower that lets us focus in on specific voices or noises amidst chaos, much like how we train ourselves to tune out background noise in a crowded room.
The researchers used electroencephalography (EEG) recordings to measure the brain activity associated with auditory attention. They found that certain parts of the brain, such as the frontal and parietal lobes, are more active when we focus on specific sounds. By analyzing these brain signals, the machine learning algorithm can learn to predict which sounds a person is paying attention to.
The study also explored the effect of segment length on decoding accuracy. Longer segments led to improved performance, much like how practicing a skill for longer periods leads to better proficiency. This finding suggests that there may be an optimal duration for training auditory attention systems to improve their accuracy.
In conclusion, this study demonstrates the potential of deep neural networks for auditory attention decoding and highlights the importance of segment length in improving performance. The researchers’ approach could have significant implications for various applications, such as speech-to-speech translation and noise reduction. By harnessing the power of machine learning algorithms, we can unlock new possibilities for understanding and interpreting complex sounds like speech.
Audio and Speech Processing, Electrical Engineering and Systems Science
Deep Neural Networks for Speech Processing: A Comprehensive Review
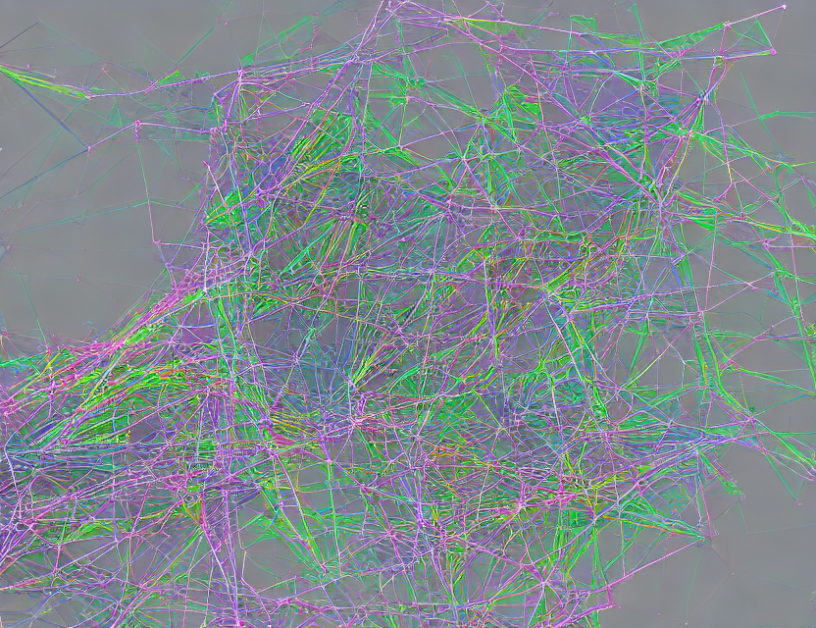