In this paper, researchers explore the use of a lightweight model for video quality assessment, which is crucial in video compression and transmission. The authors compare their proposed model with existing models, highlighting its advantages in terms of computational efficiency and accuracy. They also discuss the importance of considering different quality parameters (Qps) when evaluating video quality.
The authors begin by explaining that traditional video quality assessment models are often too complex and computationally expensive, making them unsuitable for real-time applications. To address this issue, they propose a lightweight model with only 3K parameters and 3K multiplication and accumulation (MAC). This design allows the model to be trained quickly and efficiently while maintaining accurate predictions of video quality.
The authors compare their proposed model with several existing models, including CNN-JNQD [4], which has 38K parameters and is not suitable for higher-definition input. They show that their lightweight model outperforms these larger models in terms of computational efficiency while providing similar accuracy.
The authors also emphasize the importance of considering different Qps when evaluating video quality. They explain that different Qps can have a significant impact on the perceived quality of videos, and that their proposed model can adapt to different Qps.
In summary, this paper proposes a lightweight model for video quality assessment that is efficient and accurate while being able to adapt to different quality parameters. The authors demonstrate the advantages of their proposed model through comparisons with existing models and highlight its potential applications in real-time video compression and transmission.
Electrical Engineering and Systems Science, Image and Video Processing
Enhancing Perceptual Video Coding with Deep Learning-Based JND Models
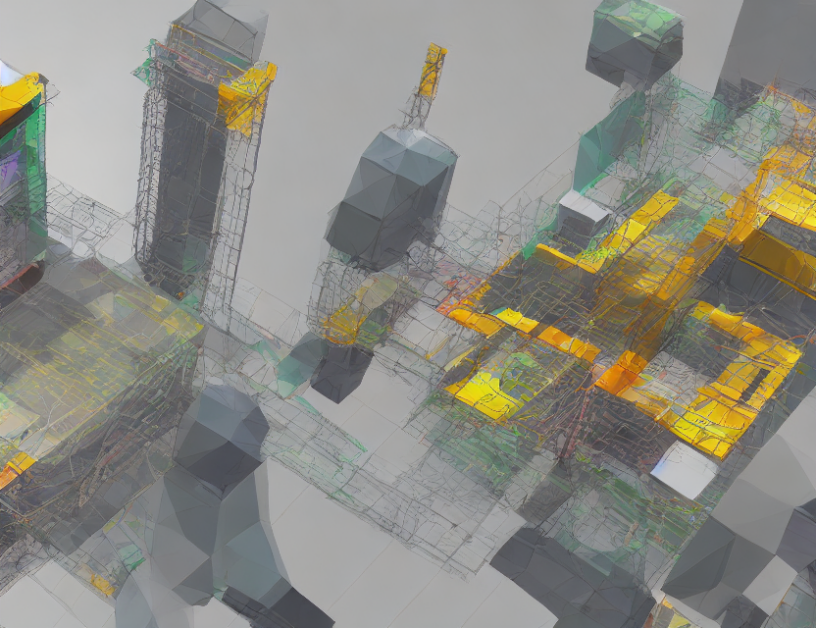