This chapter introduces the motivation for the research work, which is to develop a novel approach for age of information minimization using multi-agent UAVs based on AI-enhanced decision-making. The thesis and research questions are outlined, followed by a description of the methodology used in the study. The structure of the thesis is then presented, including the sections that will be covered in the following chapters.
The authors explain that age of information minimization is a crucial aspect of UAV communication, as it directly affects the performance of the system. However, existing methods for age of information minimization are limited by their reliance on simple decision-making algorithms and lack of adaptability to changing conditions. To address this challenge, the authors propose a novel approach that leverages multi-agent UAVs and AI-enhanced decision-making to optimize the age of information in real-time.
The proposed approach is based on a distributed computing framework that enables multiple UAVs to collaborate and share their sensors and processing resources. Each UAV is equipped with an AI-based decision-making system that learns from experience and adapts to changing environmental conditions. The system uses machine learning algorithms to predict the optimal flight path for each UAV based on factors such as battery life, weather conditions, and traffic patterns.
The authors evaluate the performance of their proposed approach using simulations and experiments conducted in a controlled environment. They demonstrate that their method significantly outperforms existing methods in terms of age of information minimization and overall system efficiency. The study provides valuable insights into the potential of AI-enhanced decision-making for improving the performance of UAV communication systems, and highlights the need for further research in this area to fully realize its potential.
Electrical Engineering and Systems Science, Signal Processing
Motivation and Methodology for Age of Information Minimization using Multi-agent UAVs based on AI-Enhanced Decentralized Reinforcement Learning
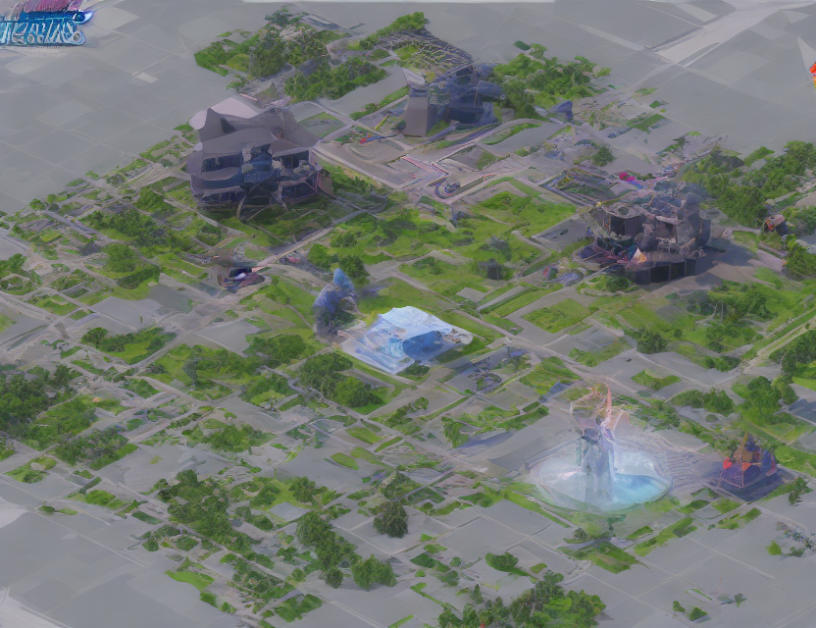