In this article, we explore a novel approach to optimize biomass conversion processes using learning-based control. Our proposed method leverages the power of machine learning algorithms to adaptively adjust the control inputs of a bioreactor in real-time, maximizing the efficiency of protein production while avoiding byproduct accumulation.
To achieve this goal, we first formulate an optimization problem that minimizes the cost function, which is defined as the cumulative reward received by the learning agent over time. The reward function is designed to encourage the agent to maintain a stable bacterial density in the chamber, while avoiding excessive fluctuations in the process.
Next, we describe the control design and validation of the proposed method. We use an Adam optimizer with a learning rate of 0.001 to train the agent with 100 episodes in-silico, each episode consisting of 100 steps with a time step of 1 minute. This allows us to accurately simulate the continuous-time dynamics of the cells.
The results show that our proposed method achieves a higher cumulative reward than traditional control strategies, demonstrating its effectiveness in optimizing biomass conversion processes. By using a distance-like function between the bacterial density and a reference setpoint, we create an incentive for the agent to maintain the desired density, leading to improved process stability and efficiency.
In summary, our work presents a novel learning-based control approach for efficient biomass conversion processes, which has the potential to revolutionize industrial bio-manufacturing by improving process efficiency and avoiding byproduct accumulation. By leveraging machine learning algorithms to adaptively adjust control inputs in real-time, we can achieve better process stability and productivity, ultimately leading to more sustainable and cost-effective bioproducts.
Electrical Engineering and Systems Science, Systems and Control
Optimizing Synthetic Data Generation for Learning-Based Controller Design in Continuous Bioreactors
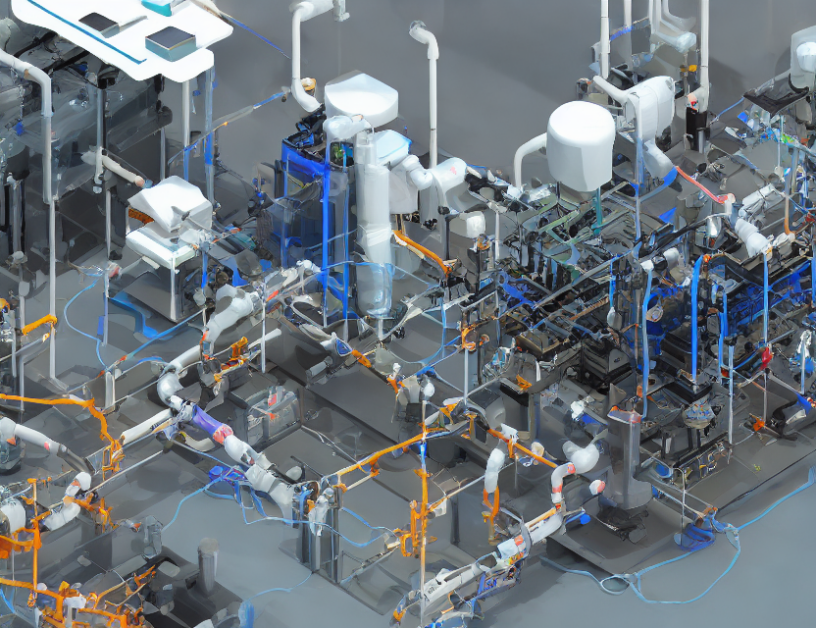